Summary
This report is the first in our Do Your Own Research series. It offers a comprehensive and generalized overview of how to use on-chain data for the fundamental analysis of cryptoassets. Later reports in this series will dive deeper into how to leverage on-chain data for evaluating specific sectors of cryptoassets including Decentralized Finance assets, non-fungible tokens, metaverse tokens, and more. In this introductory report, we present a detailed lexicon for the most popular metrics used in fundamental analysis and offer a framework through which these metrics can offer accurate and nuances conclusions about a cryptoasset’s valuation. Finally, the report offers an exhaustive record of the many iterations and innovations that have been made in this field of study, highlighting how the fundamental analysis of cryptoassets is an evolving science that is becoming more sophisticated as the adoption of cryptoassets grows.
Introduction
The study of on-chain fundamentals often focuses on measuring the intrinsic value of a cryptoasset using data derived directly from the blockchain. It differs from evaluating cryptoassets based on technical analysis (TA) or social sentiment, which attempt to measure an asset’s value by identifying patterns and trends in market activity or media attention.
The efficient markets hypothesis suggests that price should effectively reflect the realities of an asset’s fundamentals—essentially that there is no room to make excess profits in freely traded assets because the market will have already fairly priced them based on all available information. However, in practice, markets are far from perfect. Inefficiencies—and alpha—persist due to information asymmetries, transaction costs, and human emotion.
This is especially true for cryptoassets, where the nascency of blockchain data, user and developer behavior, and the asset class as a whole make it difficult to apply standardized methodological approaches to valuation. While investors have spent centuries evaluating traditional and older asset classes, such as equities, fixed-income products, and commodities, only in the last several years have sophisticated investors sought to value cryptoassets. While some traditional methodologies, like discounted cash flow or stock-to-flow, have been applied to cryptoassets, they often fall short in the face of intricacies and idiosyncrasies of blockchain-based assets.
Valuing cryptoassets relies on a hodgepodge of metrics invented over the past decade to measure on-chain activity through various lenses, many of which continue to be innovated and developed upon to this day. The most popular on-chain metrics for fundamental analysis were created for Bitcoin, the world’s first cryptocurrency, and may not be as useful or relevant when applied at face value to other cryptoassets beyond Bitcoin. Later reports in this “Do Your Own Research” series will focus primarily on the application of on-chain fundamental analysis to new and emerging sectors of the crypto industry.
Because fundamental analysis of cryptoassets relies on examining data from the blockchain, investors must develop a deep understanding of a coin’s underlying technology, meaning its core consensus or security model, before utilizing on-chain data in any valuation analysis. The use of on-chain metrics for valuation analysis must be informed by a strong knowledge of the technical functions and features of a cryptoasset and its blockchain, and researchers must be willing and able to adjust or tweak calculations to more accurately pinpoint trends impacting a coin’s intrinsic valuation.
There are several competing methodologies used to process on-chain data and aggregate them into even the most basic on-chain metrics. Understanding the strengths and weaknesses between the different approaches to on-chain data from industry-leading blockchain data providers such as Coin Metrics, Glassnode, IntotheBlock, and Dune Analytics is foundational to the application of more complex valuation methodologies such as Bitcoin Days Destroyed, Network Value to Transactions, Market Value to Realized Value, HODL Waves, Difficulty Ribbons, and more.
Rather than being a one size fits all solution to cryptoasset valuation, this report argues on-chain fundamentals are metrics to be applied dynamically and adapted frequently as the market and technology of cryptoassets also evolves. In addition, as the basis for an investment decision or a trade, on-chain fundamentals must be coupled with TA or sentiment analysis to create a holistic view on the value of a cryptoasset. This report dives into the building blocks needed to understand and correctly apply fundamental valuation methodologies for cryptoassets.
The Blockchain Data Provider Landscape
Cryptoassets are issued and transmitted by public blockchains. There are multiple ways to go about incentivizing the security, use, and maintenance of blockchains, which impacts how data is stored on these networks. Depending on the blockchain and its technical underpinnings, information about a cryptoasset issued on the network will be recorded differently. The role of blockchain data providers is to index and parse raw blockchain data into easily readable and accessible formats for users and investors.
Blockchain explorers such as Blockchair and Etherscan feature basic information about the real-time transaction history of a single coin. These explorers are public websites that can be used to verify the state of a blockchain as soon as the network is live. For many public blockchains, like Bitcoin, users can also run their own archival nodes and to capture raw blockchain data themselves. However, when it comes to analyzing usage patterns of a blockchain over time and comparing them with other chains, users normally must rely on cross-asset data providers such as Coin Metrics and Glassnode.
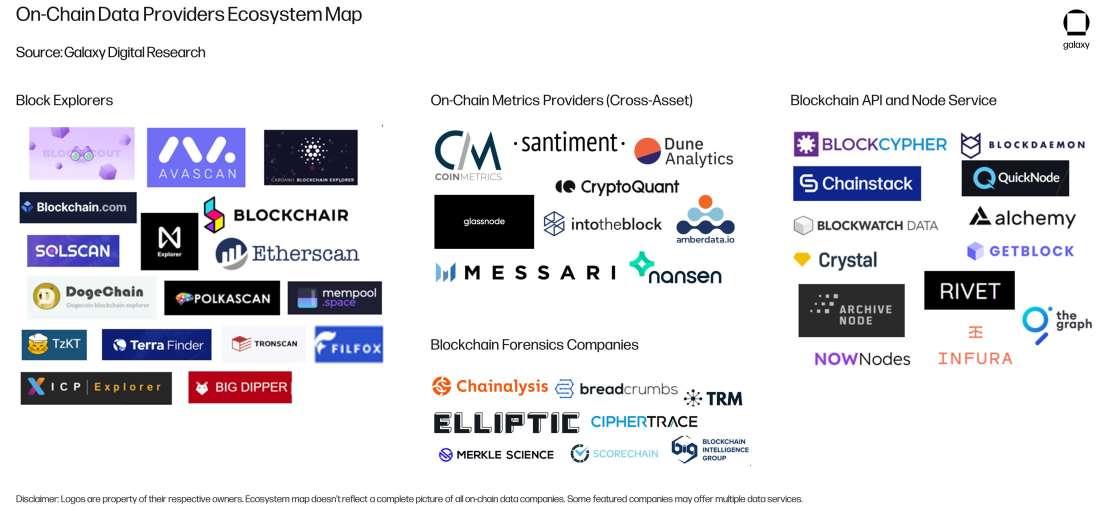
Cross-asset analysis requires a set of standardized metrics and methodologies for comparing transaction activity on one blockchain to another. Making the comparison as accurate and as fair as possible between multiple blockchains requires additional engineering effort on the part of data providers that is not required by providers when building a single asset blockchain explorer. There may be data created and stored by a blockchain or a set of blockchains that is not tracked by others due to differing network use cases.
Moreover, the methodology used to calculate a metric for one blockchain may need adjusting to capture the same activity on another blockchain. This is because not all metrics available for a single cryptoasset are easily available for another. “Transactions” on one network may represent wholly different user activity than a “transaction” on another. These are considerations that blockchain data providers need to assess when ingesting the data from a new blockchain network, and which investors much consider when comparing activity across chains. Most networks are different, and their data requires significant engineering effort to properly and consistently ingest, index, and display in a useable manner.
As such, data providers that specialize in cross-asset, on-chain analytics will likely not carry information about newly launched cryptoassets. Depending on how much data there is to ingest and how complex the information recorded, there is usually a lag between the launch of a new cryptoasset or blockchain and when on-chain metrics about the network becomes available through these providers. When a new cryptoasset is onboarded by a blockchain data provider to their existing suite of assets, there are a handful of metrics that are near guaranteed to be made available. These metrics track the basic functions of any blockchain, no matter the technical underpinnings of the network. They are metrics that track the issuance, transfer, and supply of a cryptoasset. These activities represent the core functionality of all blockchains and as such can be thought of as the baseline for using on-chain data to value cryptoassets.
Primary Metrics
Starting with a set of ten primary metrics to track the most basic functions of a blockchain, this section of the report will examine the building blocks of on-chain fundamentals. The metrics include:
Total daily issuance: The number of coins newly created on the blockchain aggregated over 24 hours.
Annual inflation rate: The number of new coins issued over the course of a year divided by the current circulating supply of coins by the end of the year.
Total transfer volume: The number of coins moved from an address.
Transaction count: The number of transactions executed on the blockchain.
Average transaction size: The mean number of coins moved per transaction.
Daily active addresses: The sum of addresses that has sent or received coins at least once over 24 hours.
Daily new addresses: The sum of new addresses appearing for the first time in a transaction over 24 hours.
Coin supply distribution: The distribution of total circulating supply across all addresses.
Total circulating supply: The number of coins issued since the genesis of the blockchain.
Supply last active: The sum of coins that has been moved to another address at least once over a given time interval.
It is important to highlight the methodologies that can be used to calculate each of the above metrics as they may significantly impact resulting values. As mentioned, the methodology may differ depending on the cryptoasset and the technical features of the underlying blockchain used to issue the asset. However, even for the same cryptoasset, there may be multiple methodologies for calculating a metric derived from different interpretations of on-chain activity.
From the metrics tracking the issuance, transfer, and supply of cryptoassets to more complex valuation models such as spent output profit ratio (SOPR), network value to transactions (NVT), and coin days destroyed (CDD)—the latter of which will be discussed in detail later in this report—blockchain data providers may use different methodologies for their metric calculations. These differences stem from a nuanced understanding of how underlying blockchains’ consensus algorithms, transaction models, and/or monetary policies work.
Issuance Metrics
Starting with metrics that measure the growth rate of a cryptoasset’s supply, the intuitive methodology is to count how many coins are newly issued on a blockchain over a given time. Tracking this data can be useful for understanding how scarce a cryptoasset is and can be coupled with other basic metrics such as the total circulating supply of a coin, to identify and highlight changes in the inflationary or deflationary trends of a coin.
One of the most famous metrics used to assert Bitcoin’s long-term value proposition as a hedge against inflation is its annual inflation rate. Bitcoin’s software is designed to automatically reduce its issuance of new coins by 50% every 210,000 blocks (roughly four years). This mechanism, also called the “halving,” is what makes bitcoin a scarce asset. Anyone can verify that bitcoin’s supply schedule (and, therefore, scarcity) remains intact by examining on-chain data. This verification can be performed several ways, including examining each block and ensuring that the miner was rewarded with the correct amount (6.25 BTC per block today), a function that bitcoin nodes conduct by default.
When we view the issuance on an aggregate and annualized basis and compare against the total circulating supply of all bitcoins, we can see the annualized rate of monetary issuance (inflation) and verify that the network is behaving as expected. Because Bitcoin’s issuance schedule is predetermined and unchanging, we can also project forward to calculate the expected issuance and inflation rate for any given point in the future.
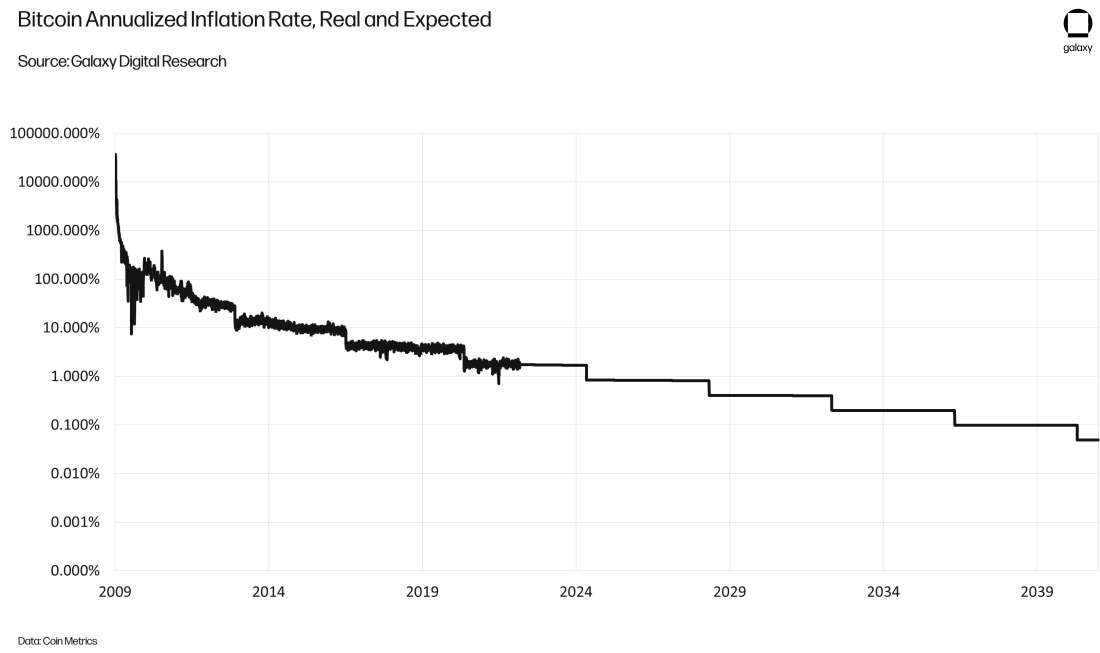
As an aside, it’s worth noting that newly minted BTC are issued when a new block is mined, rather than at a specific time. Due to an ongoing increase in hashrate, which is a measure of the collective computational energy expended from miners, Bitcoin’s halvings have historically occurred slightly sooner than expected. For example, a model calculated from genesis suggests Bitcoin’s first halving should have occurred on December 31, 2012, but in practice it occurred on Nov. 29, 2012. Bitcoin’s second and third halvings also occurred months earlier than what was expected according to the network’s theorized design.
A growing hashrate has caused Bitcoin’s mining difficulty to adjust almost exclusively upwards over the last 12 years to bring the interval between blocks closer to the target of 10 minutes. Leaving 2009 out of the dataset, as mining in the early days was sporadic, the average interval between blocks has been 563 seconds since 2010, which is about 6% faster than the target, according to analyses of data from Coin Metrics. Blocks being mined slightly faster than target results in quicker halvings and therefore faster BTC issuance.
The intuitive methodology for calculating inflation captures the scarcity of Bitcoin’s coin supply over time but it is not as useful when applied to Ethereum. To encourage a gradual transition away from proof-of-work (PoW) mining entirely, developers have created a mining “difficulty bomb” on Ethereum which periodically increases the difficulty of mining (and therefore reduces the issuance rate). The efficacy of the difficulty bomb on Ethereum is hotly debated as developers have had to delay the impacts of the bomb for multiple years now due to a lack of preparation and readiness for the network’s transition away from PoW.
Last August, Ethereum developers introduced a protocol upgrade that dramatically altered the issuance dynamics of its native cryptocurrency, ether, by introducing a burn mechanism to destroy previously issued coins from circulation. The code change, known as Ethereum Improvement Proposal (EIP) 1559, is aimed at improving the user experience when determining an optimal transaction fee, especially during times of congestion and high transaction activity. Among other updates like increasing Ethereum’s block size, EIP 1559 removes a portion of ether known as the “base fee” spent in transaction fees. For more information about EIP 1559, read our report on the impacts of the upgrade here.
Using the same methodology for inflation as Bitcoin (the total issuance of new coins divided by the total circulating supply), the scarcity of ether does not appear to have deviated from pre-EIP 1559 levels. However, by factoring in coin burns as a variable, which offset the total issuance of new coins, the values for the inflation rate of ether changes and shows a decline from roughly 5% per annum down to 2%.
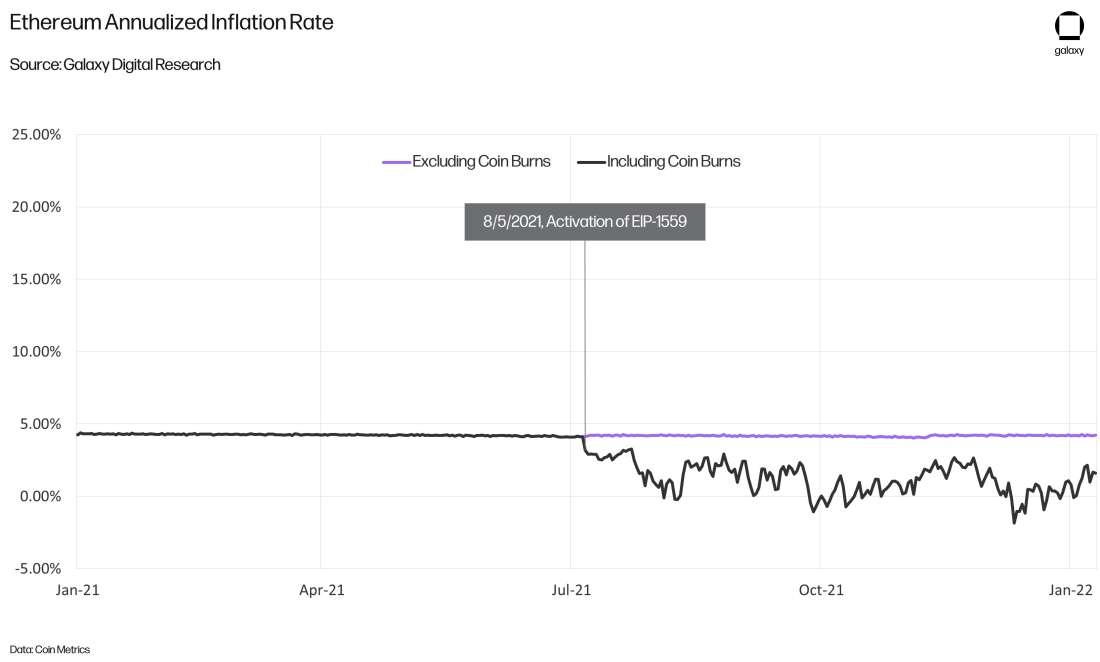
Major data providers Coin Metrics and Glassnode calculate annualized inflation for Bitcoin and Ether without incorporating coin burns as an offsetting force to daily issuance. This is an unintentional consequence of using a standardized methodology that can be easily and uniformly applied to multiple cryptoassets. It is simpler on the part of blockchain data providers to apply the same calculations across blockchains rather than introduce variances that incorporate the nuances of every network’s underlying protocol. This is a perfect example of why researchers must take an asset-specific view, even when evaluating common metrics like inflation rate.
Using such a generalized methodology on Ethereum illustrates the unchanging upper bound for the inflation rate of ether based strictly on the issuance of new coins and the total coin supply. However, it does not accurately reflect changes in the net growth rate of ether’s supply because of the burn mechanism of EIP 1559. Introducing coin burns—to the extent they exist on a blockchain—into the methodology for annualized inflation is one of the ways investors can better evaluate changes in the scarcity of ether resulting from elevated on-chain activity and ensuing high transaction fees.
Transfer and Transaction Metrics
The importance of methodology when it comes to applying fundamental metrics is even more pronounced when measuring the frequency and volume of on-chain transactions.
The main motivation for tracking transaction count and transfer volume is to ascertain trends in the usage of a coin, especially deriving the real economic activity of a coin’s users. By tracking how frequently a cryptoasset is exchanged and at what volume, investors can begin to measure the underlying network’s usage patterns. The difficulty when it comes to measuring transaction activity is identifying which transactions are representative of economic activity and which are not. Due to the differences in how blockchains send and receive transactions, and what constitutes a “transaction,” the methodologies used to filter out meaningless transactions and volume will vary.
The following are three different ways transfer and transaction metrics can be calculated on Bitcoin and Ethereum.
Grouping Transactions by Type
Change and Coinbase Transactions
For Bitcoin, there are two types of on-chain transactions that are identified by most data providers as being unrepresentative of real user activity. Coinbase transactions are usually excluded from metric calculations as they represent automatic transfers of newly issued bitcoin from the protocol to a miner as opposed to a transfer of bitcoin from and to a user. Note that this terminology does not refer to transactions made on Coinbase, the cryptocurrency exchange. Generally, Coinbase, the exchange, is referenced by using a capital “C” and coinbase, the issuance of the bitcoin blockchain, is differentiated using a lower case “c”.
The second type of transaction on Bitcoin that is usually excluded from transaction metric calculations is change outputs. When a Bitcoin user sends bitcoin to a recipient, the user’s wallet software selects from several available pieces of coin (unspent transaction outputs, UTXOs) and uses as inputs to the transaction enough pieces of coins to satisfy the transfer, returning any excess amount to the sender’s wallet as a new piece of coin. The returned, excess amount is referred to as change. (As an aside, software that helps users manage the private keys to multiple blockchain addresses on behalf of a user is known as a cryptocurrency wallet.)
It is considered best practice on Bitcoin to have change outputs sent to a new bitcoin address, as opposed to the same sending address. This is to protect user privacy and ensure a user’s transaction history cannot be linked to a single blockchain address. Given that change outputs are not representative of new transfers of volume initiated by users and instead are transactions that restore the balances of users after their transfer is complete, this type of transaction, when identifiable, is normally filtered out from the metric of transaction count and transfer volume by analysts and on-chain data providers. Simply, it’s not a transaction, it’s just how Bitcoin works.
Excluding known change outputs that are sent back to the same originating address can significantly alter the resulting values for transaction and transfer metrics. This is evidenced by comparing metric values between data providers Coin Metrics and Glassnode. On Glassnode, unadjusted transfer volume counts only excludes coinbase transactions while including all others. On Coin Metrics, the unadjusted transfer volume automatically excludes known change outputs. This minor difference in methodology can impact values significantly, overstating them by more than 50x on certain days.
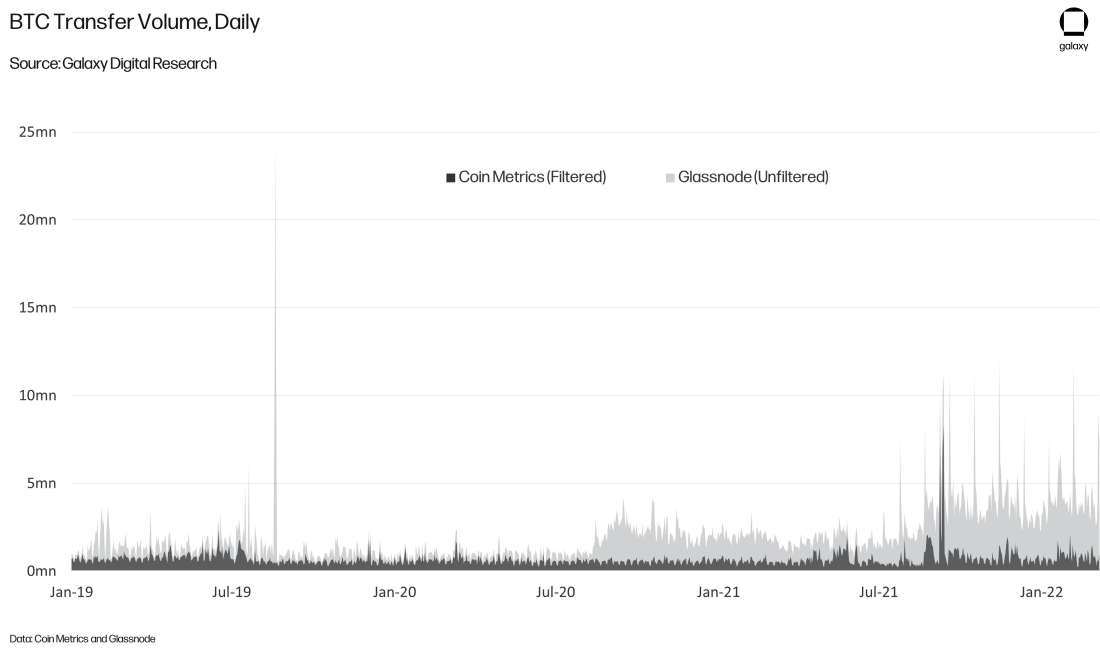
On Glassnode, there are several filtered versions of the transfer volume metric that does offer the same adjustments as the ones automatically applied by Coin Metrics. For example, Glassnode offers a separate version of the metric that is adjusted for change outputs and an additional version that adjusts for address clusters, which are addresses identified through advanced heuristics and techniques to likely be the owned by the same user. Coin Metrics also offers multiple variations to their base transfer volume metric, which will be discussed in more detail later in this section of the report.
OP_RETURN Transactions
On-chain transactions that are an automatic byproduct of the day-to-day operations of a blockchain network are notorious for inflating metrics on newer blockchains. On such example is Solana, which records gossip between validator nodes as “transactions” on its blockchain. To avoid misleading conclusions about the utility of a chain and, by association, the value of its native cryptoasset, it is important to consider the types of activity considered to be “transactions” by a blockchain network, as well as what type of cleaning and filtering of this data is being performed by on-chain data providers. Being aware of these intricacies, or removing this data entirely, is important for filtering out non-economic transaction activity or helping to identify different types of blockchain usage.
Going back to Bitcoin as an example, there are transactions that users can initiate and pay for through fees that will not send BTC but rather will store arbitrary data on-chain. The flexibility of the Bitcoin protocol in storing arbitrary data is limited and does not compare to the more composable virtual machines of general purpose blockchains like Ethereum, Avalanche, Solana, and the like. However, as the most decentralized and therefore censorship-resistant blockchain in the world, Bitcoin can execute what are called OP_RETURN transactions which allows users to take advantage of the network’s immutable ledger of transactions to record their own messages.
These messages can include reference data for second-layer protocols (like the Omni Network, upon which the stablecoin Tether was first issued), or any arbitrary data like links, hashes, plain text, or even images. In addition, OP_RETURN transactions have been used as a mechanism to timestamp arbitrary data and make it easy to independently verify when data was created in the past. OpenTimestamps and OriginStamp are two protocols that rely on the timestamping native to Bitcoin blocks and commit hashes of arbitrary data to these blocks. Given the variety of use cases for OP_RETURN transactions, there are a few Bitcoin blockchain data services that strictly track these types of transactions. For example, the OP_RETURN bot on Twitter writes a tweet every time a new OP_RETURN transaction is committed on-chain.
In theory, any amount of data can be stored in an OP_RETURN transaction. However, most nodes and miners will not accept an OP_RETURN transaction greater than 80 bytes. Most OP_RETURN transactions store far less than the maximum amount and therefore on average have been cheaper to execute on Bitcoin than other types of transactions. They were initiated in high volumes in late 2018 following the launch of VeriBlock, a separate proof-of-proof network that stores records of its own blocks as arbitrary data on Bitcoin’s blockchain to increase its security.
Knowing this, the surge in transaction count from late 2018 to the middle of 2019 can be easily identified as having been fueled in part by OP_RETURN transactions as opposed to peer-to-peer transfers of bitcoin. As evidenced in the chart below, more than 70% of all OP_RETURN transactions were predominantly transfers of USDT on Bitcoin in June 2019.
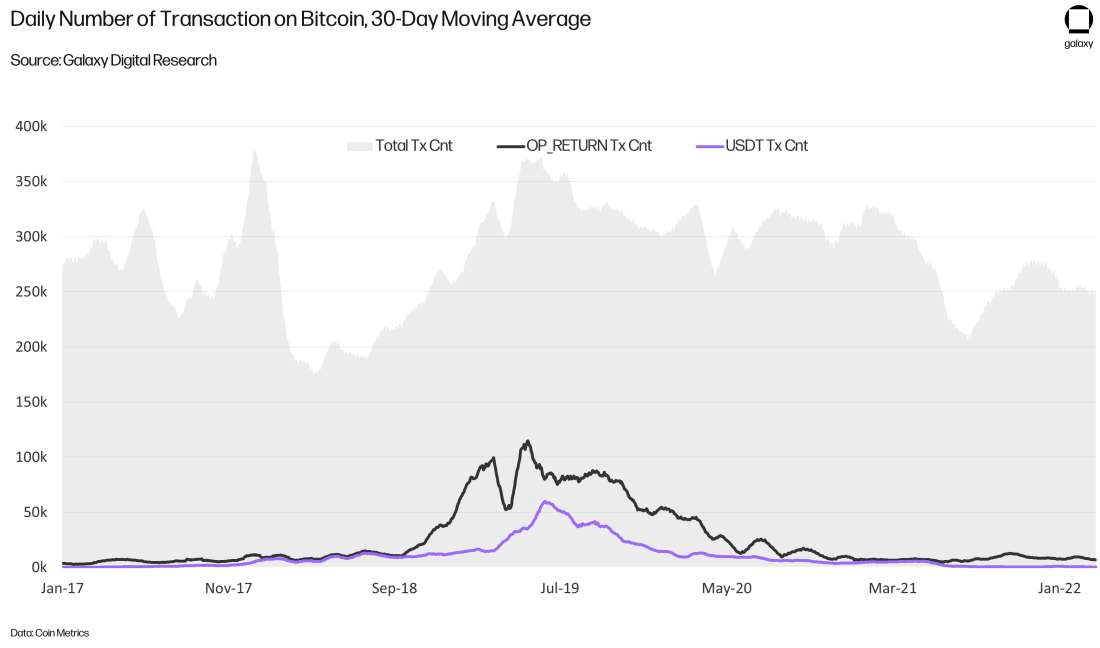
It’s worth noting that on Bitcoin, transaction activity representing real economic activity can also be undercounted through on-chain transaction and transfer metrics, rather than simply over-counted by the inclusion of OP_RETURN and coinbase transactions or change outputs. This because well-known cryptocurrency exchanges such as Coinbase and mining pools are known to use a technique called transaction batching. A batch transaction is one in which one transaction spends coins (contains outputs) to many different individuals. Batching is often used by exchanges to reduce the number of transactions, amount of block space, and ultimately fees required to process multiple user withdrawals. Bitcoin’s UTXO model enables a single unspent output of BTC to be paid out in parts to multiple addresses. While each transfer of BTC may represent a transaction to a unique party, on-chain data will record each transaction as one even if it functionally includes multiple transfers to various parties, thereby undercounting the real economic activity occurring on the network.
ERC-20 and ERC-721
Another example of how transaction types can be useful for explaining trends in the utility of a blockchain is seen on Ethereum. Most non-ether cryptoassets traded on Ethereum, also called tokens, are coded according to an interoperability standard. While there are several standards, the most widely used token standard on Ethereum is called the ERC-20, which is a model for creating fungible tokens. However, more recently, two alternative token standards, ERC-721 and ERC-1155, have been rising in popularity as trading activity around non-fungible tokens (NFTs) has taken off on Ethereum. Analyzing the transaction activity of ERC-20 versus ERC-721 and ERC-1155 tokens illustrates how the primary use case for Ethereum has begun to shift.
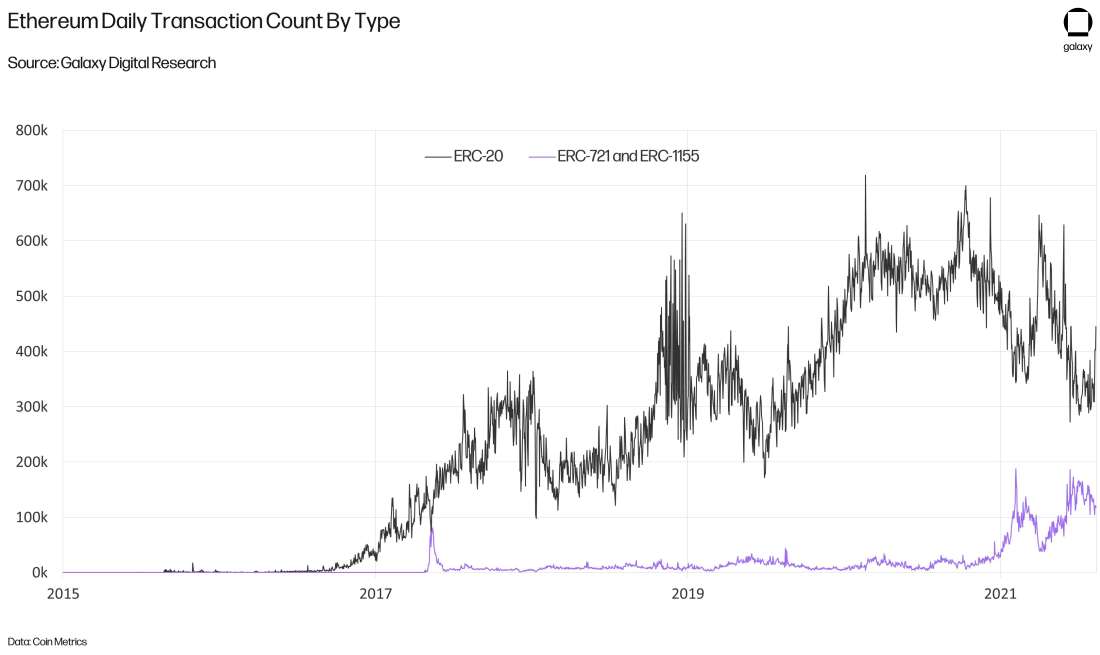
Grouping Transactions by Behavior
Outside of transaction type, transactions can also be grouped by behavior and value. The main benefit of grouping by behavior is to exclude repetitive transactions of coins sent and received by the same user from metric calculations. Cryptocurrency exchanges often aggregate user deposits and sweep these coins from hot wallets (a wallet that is connected to the internet) to cold wallets (wallets typically stored on physical devices that are “air-gapped,” i.e., not connected to the internet) for longer-term storage. In addition, coins may intentionally be moved through multiple addresses to obscure the origin of funds and enhance the privacy of transactions. Coin mixers are applications on public blockchain like Bitcoin and Ethereum that will do this automatically on behalf of users.
Coin sweeping behavior can inflate transaction metrics by making it appear as if each transfer is a new transfer of value between distinct users. To account for this behavior, data providers such as Coin Metrics perform additional filtering to generate “adjusted” versions of transaction count and transfer volume. These adjusted metrics do not count each subsequent and complete transfer of coins made within a four-block period (roughly 40 minutes) as separate on-chain events. Instead, the movement of coins from the first and last address identified within the period is counted as one single economic transaction. The purpose of this additional filtering is to discount full sweeps of funds from a hot wallet to a cold wallet, or additional structuring that appears programmatic rather than economic. As applied to Bitcoin, the alternative methodology for adjusted transfer volume reduces daily values on average by 770,000 BTC.
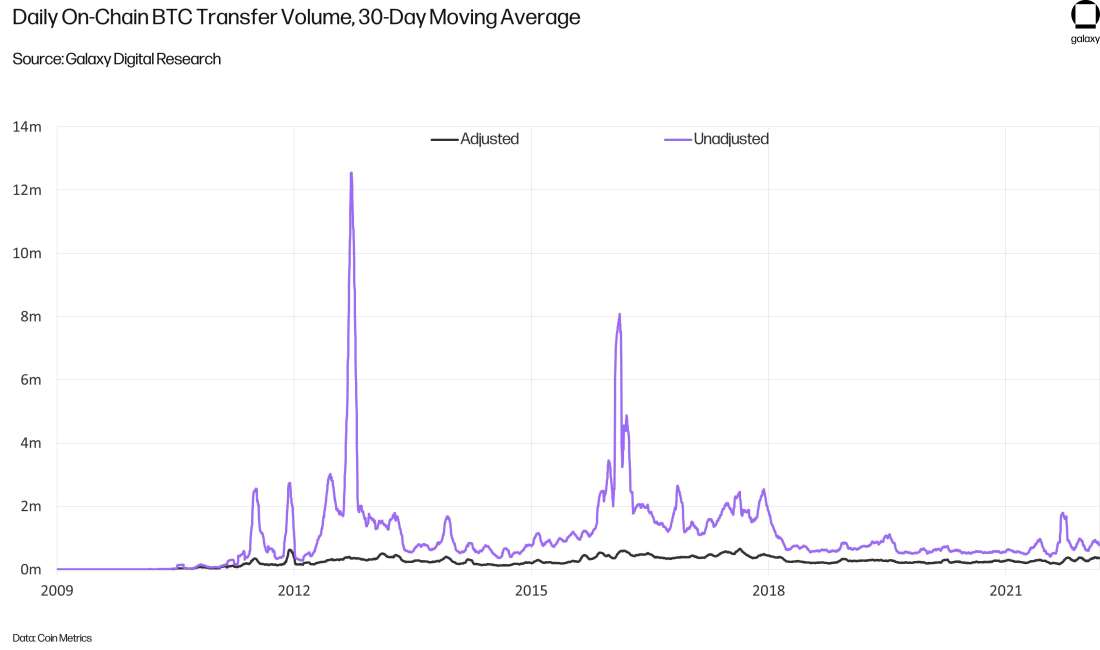
Filtering out this type of data may not always be helpful to fundamental analysis. This is because, unlike change outputs, every hop in a transaction does require the payment of an additional transaction fee, meaning there is some amount of economic value associated with each transfer that may be valuable to measure in some cases. After all, the function of fees on public blockchains is gatekeeping to prevent meaningless transactions from filling up block space. Thus, by definition, while sweeps to cold storage or intentional programmatic structuring may not be useful to measure the economic use of the blockchain, the transactions themselves cannot be said to be non-economic to the system as a whole.
Grouping Transactions by Value
In practices, fees are a double-edged sword when it comes to encouraging meaningful economic activity on-chain. Fees that are too high on a network can become barriers to entry for regular users, discouraging adoption of a cryptoasset and its underlying protocol. This has been the case on Ethereum where fees have skyrocketed over the past 2 years due to a limited block capacity and an ever-growing demand for block space. Increasing fees have made it more cost-prohibitive to send transactions of zero value on-chain. At the same time, high fees on Ethereum have also driven away real users and been the impetus for the creation of a slew of other alternative blockchains such as Solana, Avalanche, and Polygon, each boasting significantly lower, and in some cases even non-existent, fees. On the other hand, higher fees have also spurred the accelerated development of scaling solutions like Layer 2 networks and sharding, which is expected to be an important component of Ethereum’s forthcoming ETH 2.0 upgrade. And, because of the EIP-1559 upgrade, higher fees also make Ethereum more disinflationary, contributing positively to ether’s scarcity.
When it comes to these other general purpose blockchains, it is important to note that while these chains do boast higher transaction counts than Ethereum, they also attract a larger number of spam transactions due to a low barrier of entry for sending transactions. As such, raw transaction count is not usually a meaningful metric to evaluate when comparing the economic activity between Ethereum and these chains especially when the costs to use each network differs significantly. Alternatively, there are methodologies that group transactions by value and thereby intentionally exclude transactions above or below a specified threshold for the purposes of more accurately tracking the real economic activity of a blockchain.
Grouping transaction by value is a common methodology used on Bitcoin to differentiate between types of economic activity on-chain. Transactions that transfer less than $100 dollars of bitcoin is often seen as a proxy for the payments use case of the network, whereas transactions that transfer more than $1mn is viewed as measuring of the trading and investment activity of deep pocketed players (such as institutional investors). The following chart illustrates how the economic activity on Bitcoin has shifted over the last four years as the number of smaller retail transactions has declined and larger institutional trading activity has grown.
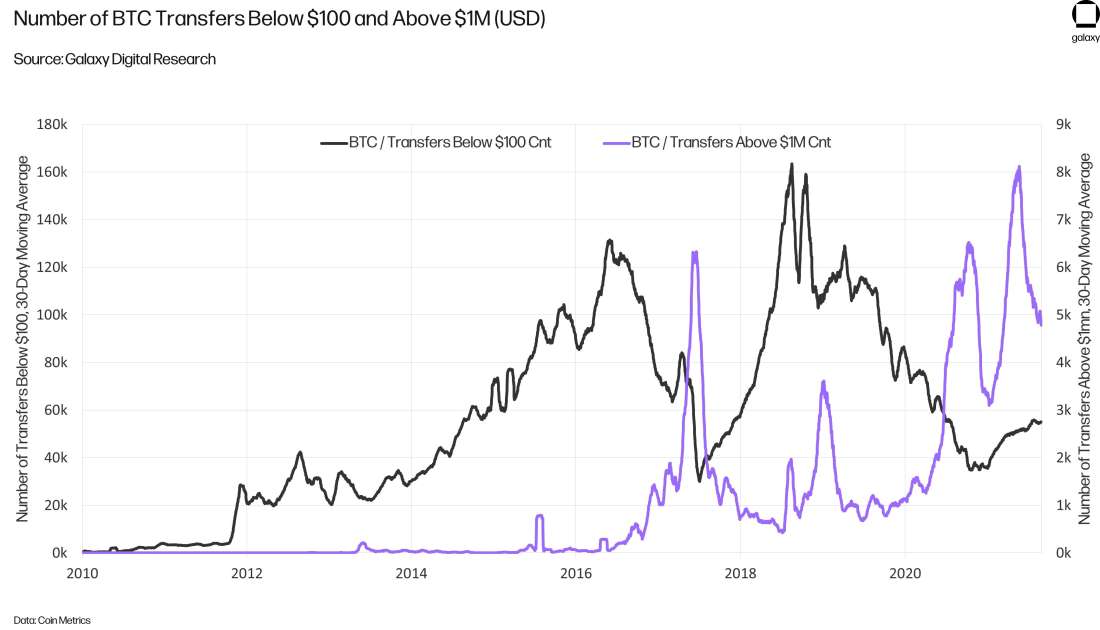
To better track economic activity on-chain, transactions can be filtered or grouped by type, behavior, and value. Determining which methodology to use and what transaction activity to include or exclude depends heavily on how the underlying blockchain is programmed to work. None of the above methodologies can be used to evaluate the economic activity of all cryptoassets but instead these methodologies should be considered case studies that inform the researcher which questions to ask when applying transfer or transaction metrics to a broader fundamental or valuation analysis.
Supply Metrics
Supply metrics are often used in conjunction with issuance and transfer metrics to serve as the building blocks for creating more complex valuation models, many of which will be highlighted in the next section of this report. On their own, supply metrics can be useful for identifying trends between long-term and short-term holders of a cryptoasset. In addition, changes in the supply of a cryptoasset held by entities such as an exchange, mining pool, or custodian can also be tracked by identifying patterns in spending and through using blockchain heuristics to label blockchain addresses.
In its simplest form, the total supply metric of a cryptoasset measures the number of coins issued to all addresses on the blockchain. This is not equivalent however to the total supply available in the market for holders to buy and sell. Issued coins may be burned, lost, locked, or otherwise taken out of circulation. They may be vesting or staked on-chain and therefore immovable for a specified period. As is the case with Bitcoin, almost 20% of total coin supply is estimated to be irretrievable due to forgotten or accidentally deleted seed phrases, or lost belonging to Bitcoin creator Satoshi Nakamoto (who is only known to have spent coins once, in the network’s first transaction, which Satoshi sent to famed cryptographer Hal Finney).
There are several reasons why the total supply of a cryptoasset may not accurately reflect that asset’s available supply. As such, data providers may offer alternative methodologies for calculating coin supply that filter out units that have a high likelihood of being temporarily illiquid or permanently lost. Coin Metrics coined the notion of free float supply, which removes coins that haven’t transacted in a long period of time, or are otherwise known to be destroyed, from the total supply. When applied to the top five cryptoassets by market capitalization, free float supply suggests that between 2% to 60% of total supply is unavailable to the market. (Note: The following table excludes Binance Coin (BNB) which Coin Metrics does not offer supply metrics for although it is among the top five cryptoassets by market capitalization.)
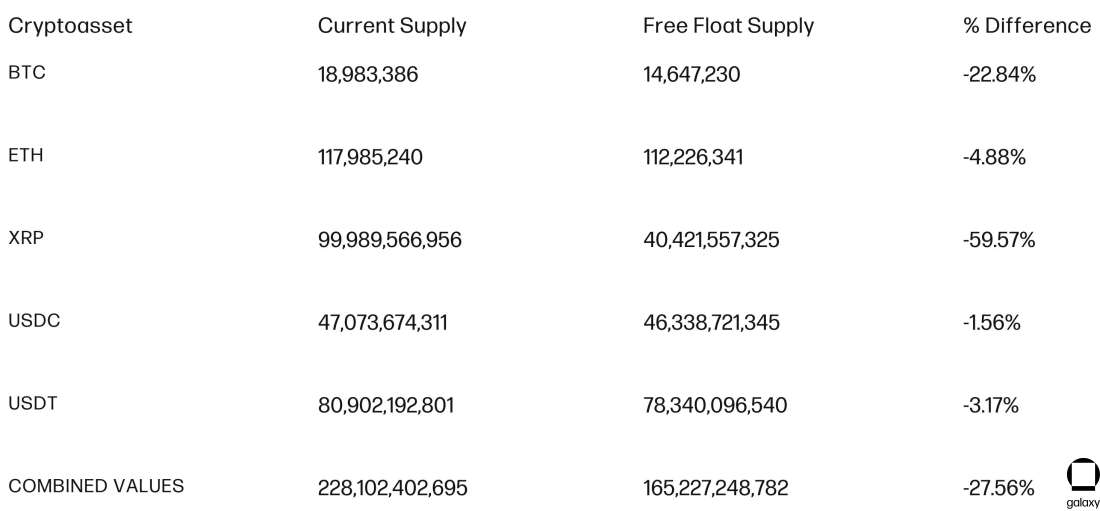
Source: Coin Metrics, Data as of March 14, 2022.
Said another way, at current prices, the total market capitalization of the top five cryptoassets is $1.2tn, but when adjusted for free float supply, that market capitalization reduces to roughly $1tn.
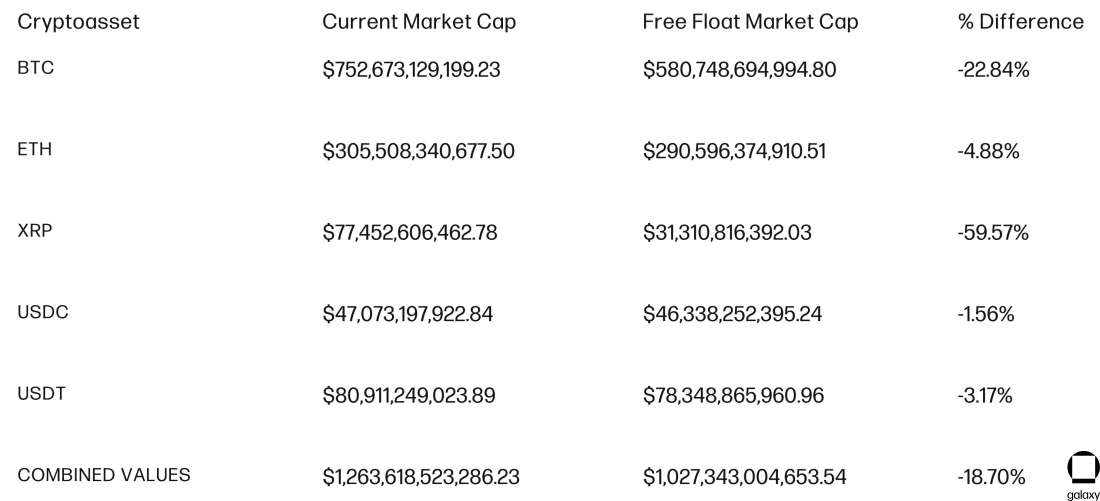
Source: Coin Metrics, Data as of March 14, 2022.
Another methodology for filtering out illiquid supply is to categorize all known blockchain entities and addresses by their historical transaction activity. This entity-based adjustment was developed by Glassnode. As background, entities are defined as bundles of addresses that are labelled and identified as being managed by a single user. Instead of tagging units of supply, Glassnode groups together the holdings of entities based on transaction history. Entities are categorized into three buckets depending on the frequency of their on-chain transactions. For example, the coin holdings of exchanges would be labelled as highly liquid while the holdings of foundations or long-term investors would be labelled as illiquid.
Using Glassnode’s methodology, the supply of liquid coins on Bitcoin is 4 million, which is significantly lower than the free float supply value recorded by Coin Metrics. The stark difference in value created from these two methodologies for measuring coin supply highlights again variations in the interpretation of on-chain data. Coin Metrics uses a simpler approach that looks primarily at the transaction history of individual units of bitcoin, whereas Glassnode for their liquid supply metric makes additional assumptions about the liquidity of coins based on entity type.
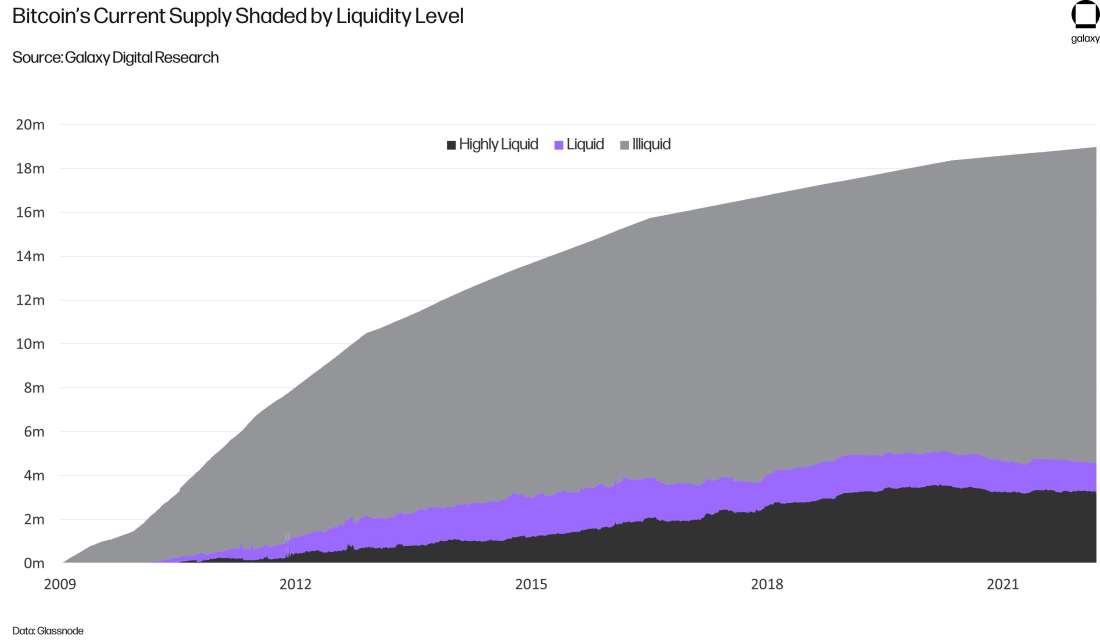
One of the advantages of CoinMetrics’ methodology is that their approach is easier to standardize and replicate across multiple blockchains as it does not heavily rely on tagging and grouping addresses for various chains. Glassnode’s entity-based approach due to its reliance on labelling all addresses which requires more on-chain analysis and proprietary machine learning software is currently only available for Bitcoin. Glassnode’s methodology and technology is also proprietary and harder to evaluate objectively.
As such, be it with the issuance, transfer, or supply of a cryptoasset, there exists variations in the methodology behind calculating these basic on-chain metrics. For investors, determining which methodology to apply requires a value judgement based on the cryptoasset in question and the data provider being relied on. These value judgements are what enables investors to come to nuanced conclusions about the trends identified through on-chain analysis. They are also what informs the creation and ongoing iteration of more complex valuation models such as Bitcoin Days Destroyed, Network Value to Transactions, and Market Value to Realized Value.
Applying and Combining the Primary Metrics
Now that we have a basic understanding of using on-chain metrics for fundamental analysis, the next step in creating valuation models for cryptoassets is to combine metrics and apply them as variables within formulas, as opposed to evaluating them as standalone calculations. In this report, we will discuss five complex valuation metrics that were created to evaluate the market value of bitcoin and determine whether BTC price is over or undervalued. They include Bitcoin Days Destroyed, Network Value to Transactions, Market Value to Realized Value, Spent Output Profit Ratio, and Difficulty Ribbons.
The Limitations of OCF for Predicting Price
At most, these valuation metrics are useful reference points for market highs and lows. They should not be relied on for any degree of accuracy in predicting or prescribing market movements. In addition, they should be distinguished from valuation metrics that seek to derive a cryptoasset’s intrinsic or “fair” value. Determining an asset’s intrinsic value relies heavily on identifying the asset’s primary investment narrative and subsequently, its perceived total addressable market.
For example, viewing bitcoin’s investment narrative as that of digital gold suggests the value and growth trend of bitcoin should eventually mirror that of physical gold. While bitcoin certainly does not behave like gold today, the exercise in comparison emphasizes the potential for what bitcoin could behave like in the markets over time. As such, comparing bitcoin’s market capitalization to that of gold’s market capitalization using on-chain metrics is one way to evaluate the intrinsic value of the asset.
There are several narratives surrounding bitcoin from a venture-investing perspective beyond digital gold including that of a global payments network and revolutionary technology akin to the internet or mobile phones. According to these narratives, comparing the growth of active on-chain addresses with the adoption curve of mobile phone users or microwave purchases is another methodology for evaluating bitcoin’s fair value. Investors could also compare the annual total BTC transfer volume as a percentage of its year-end market capitalization and compare it with that of existing payments giants such as PayPal or Mastercard.
Using on-chain metrics to support analysis into the fair value of cryptoassets requires a value judgement on what investors perceive cryptoassets to one day be worth and/or used for. Due to the novel features of this entire asset class, it can be difficult to determine what the total addressable market for a cryptoasset is or one day should be. Investors can also rely on on-chain metrics to evaluate present market sentiment and trends. At times, applying such analysis may also require a similar value judgement on an asset’s primary investment narrative, as in the case of NVT and its related metrics, but by and large, the type of analysis which aims to identify market tops and bottoms relies primarily on identifying and isolating the behavior of known network stakeholders.
As an area of study, on-chain fundamental analysis into market cycle signals has centered primarily around the world’s oldest and most valuable cryptoasset: Bitcoin. However, the growing value and investor interest in alternative cryptoassets over the years is an indicator that more complex valuation metrics evaluating the price behavior behind these assets will thrive beyond bitcoin-focused metrics. In this section of the report, we will discuss to what extent investors can apply five complex on-chain metrics to alternative cryptoassets and the effectiveness of doing so for evaluating price behavior beyond that of bitcoin.
As an evolving science, fundamental analysis is one lens through which to consider a cryptoasset’s value, but it is by no means the only lens. There are limits to how effective on-chain data can be in explaining a cryptoasset’s market value, especially when excluding other types of analysis such as social sentiment or technical analysis, particularly given the nature of cryptoasset market participants, many of whom are retail investors who trade and accumulate based on community identity. As such, the following analysis will illustrate how investors can approach fundamental analysis as a tool that can be applied and iterated upon.
Bitcoin Days Destroyed (BDD)
Starting with the earliest known complex on-chain metric, Bitcoin Days Destroyed was ideated in 2011 by pseudonymous Bitcoin user “ByteCoin.” It tracks the transaction volume of Bitcoin by weighting the movement of coins by age (i.e., by the time since the coin was previously spent). Sharp changes in BDD are indicative of dormant coins reviving and being transacted, which investors may also view as a proxy for selling behavior by long-term holders of Bitcoin.
The methodology for calculating BDD is: BTC volume transferred * Days since last movement.
Historically, spikes in BDD have been correlated with run-ups to market peaks when selling activity from old buyers to new buyers is high. Said another way, long-term holders typically begin taking profits as the price of coins rises dramatically. These events are visible during each of Bitcoin’s all-time high runs. However, investors must be careful not to misinterpret movements in this metric if the reason for dormant coins moving is a known seizure by government authorities of previously stolen bitcoins. This has been the case for the 4th and 7th largest movements in BDD, when the U.S. government transferred coins into their control after uncovering the operators of online marketplace Silk Road and the hackers of cryptocurrency exchange Bitfinex in 2020 and 2022, respectively.
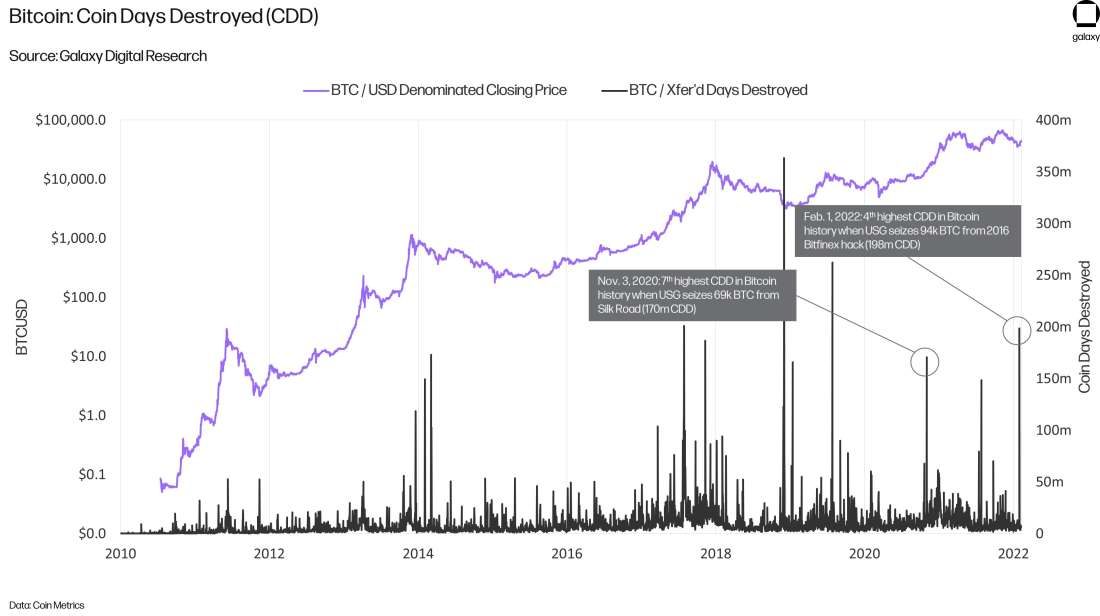
Modification #1: Changing the Building Blocks
By utilizing our knowledge of how data providers modify basic transaction metrics, we can adjust the methodology of BDD for the purposes of more accurately identifying real economic activity. For example, an entity adjusted BDD metric will only count the transfers of Bitcoin that users have sent to distinct entities, meaning blockchain addresses not controlled by the same individual or business. Glassnode offers this metric and uses the same technique for grouping addresses as the one mentioned above for calculating liquid and illiquid supply.
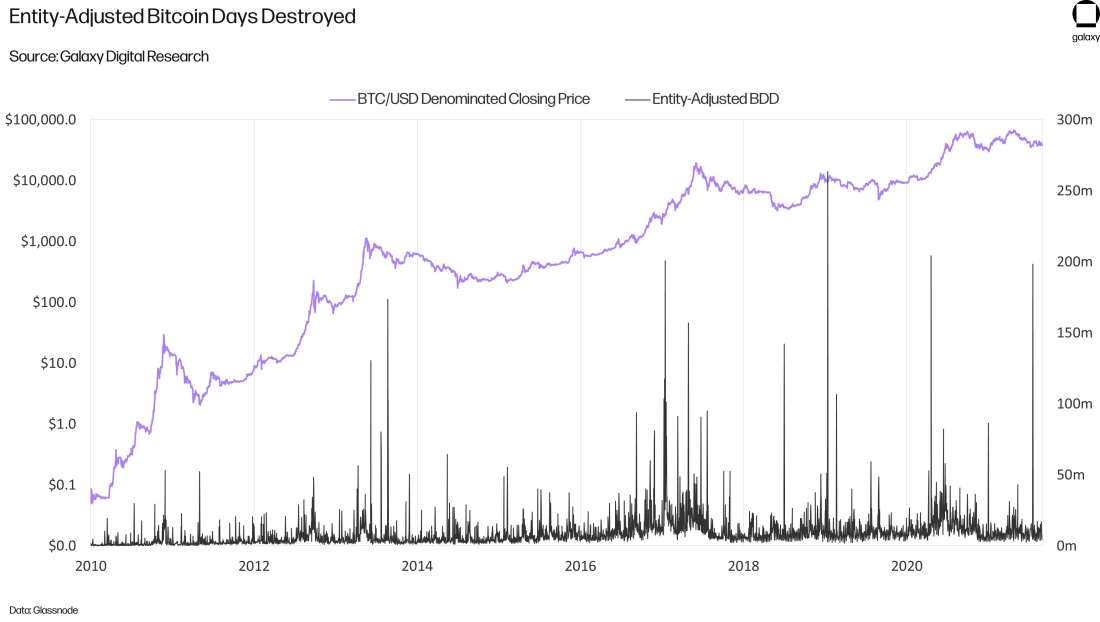
There is also a supply adjusted BDD metric which will divide BDD by the circulating supply of coins to account for the increasing scale and lifespan of old coins relative to the new ones being issued. A binary BDD evaluates whether the BDD of bitcoin each day is above or below the average BDD of prior days. Each of these methodologies makes minor adjustments to the calculations for BDD which impacts the metric’s nominal values and can more accurately highlights real economic activity.
However, none of these methodologies filter out non-economic activity perfectly and all of them record the same peaks in BDD, even the ones caused by known government seizures of BTC. As such, alternative methodologies for calculating BDD are not always useful in practice as they illustrate the same trends in coin movements, even the movements that are known to be unrepresentative of long-term holder behavior.
Modification #2: Alternative complex valuation metrics
Outside of variations to the building blocks of BDD, there are a slew of other complex valuation metrics focusing on tracking the age of cryptoassets that are based on the same concepts as BDD. While these metrics also do not filter out non-economic activity perfectly, they do offer alternative views that may be more helpful for investors to evaluate changes in the lifespan of coins over a given period. The infamous HODL waves chart is an iteration of BDD that strictly looks at the age of all issued coins in Bitcoin’s supply. It was first introduced by Jon Ratcliff in November 2014 and further developed by Dhruv Bansal of Unchained Capital in April 2018.
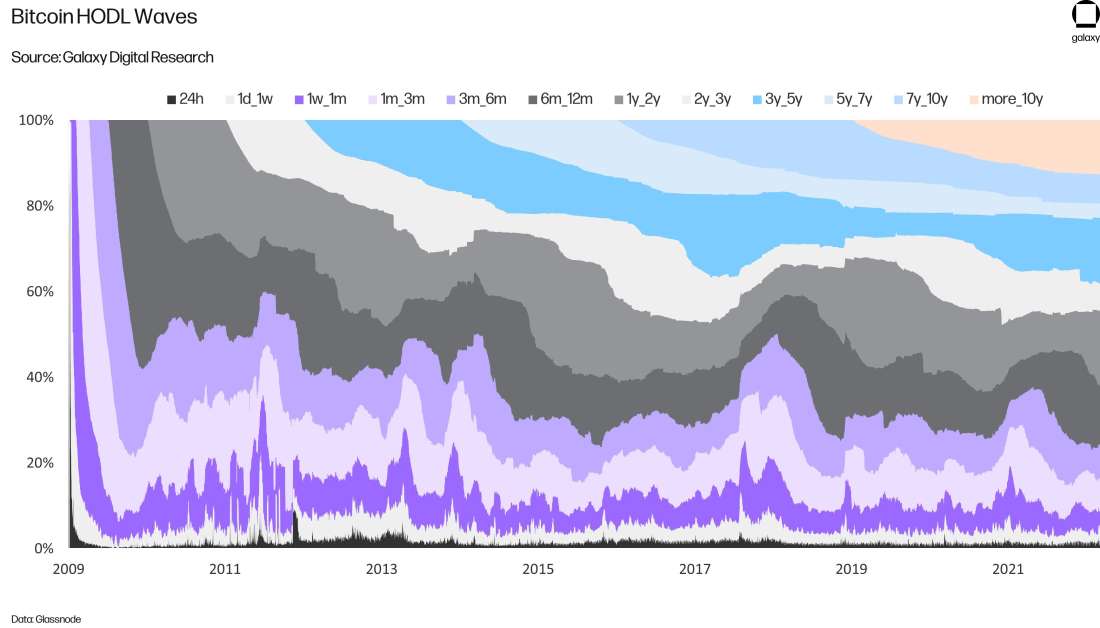
The concept for BDD has also inspired the liveliness metric, which ranges between 0 and 1, increasing as long-term holders liquidate their positions and decreasing as holders accumulate new coins. Liveliness measures the saving, as opposed to spending, activity of long-term holders.
The methodology is: (BTC transferred * Days since last movement) / (Total Coin Supply * Days since created).
Given that the basic building blocks for calculating liveliness, along with HODL waves and BDD, also exist for cryptoassets beyond bitcoin, data providers such as Glassnode offer these complex metrics for alternative blockchains such as Ethereum. For this reason, the BDD metric is frequently referred to by its more generic name, Coin Days Destroyed (CDD). However, when it comes to a network like Ethereum where users initiate transactions not only to transfer value but also to interact with smart contracts, a sudden movement of coins could be indicative of diverse types of economic activity, not only the buying or selling of coins. Even so, CDD, HODL waves, and liveliness can be useful tools for investors to gauge the activities and therefore market sentiment of long-term versus short-term coin holders when used in conjunction with other off-chain signals such as exchange activity or social sentiment.
Another on-chain variation of CDD that compares near-term spending behavior to average spending behavior over the course of a year is the Value Days Destroyed Multiple. It was proposed in November 2021 by host of the Alpha Beta Soup YouTube show and former lead bitcoin analyst at Glassnode, TXMC. metric multiplies CDD with the market price of a coin as the basis for measuring spending activity. It then divides the 30-day moving average of this figure with the 365-day moving average to identify deviations more clearly in average spending behavior. Finally, to normalize for the rise in coin supply over time through block issuance, the metric is multiplied by the ratio of current supply to future supply cap.
The methodology for calculating the Value Days Destroyed Multiple is: (30-Day Moving Average (CDD * Price) / 365-Day Moving Average (CDD * Price))* (Current Supply / Fixed Supply Cap).
As applied to Bitcoin, the Value Days Destroyed Multiple when it has registered values above 2.9 has historically indicated market peaks, while values below 0.75 has conversely indicated bearish market sentiment and low market activity.
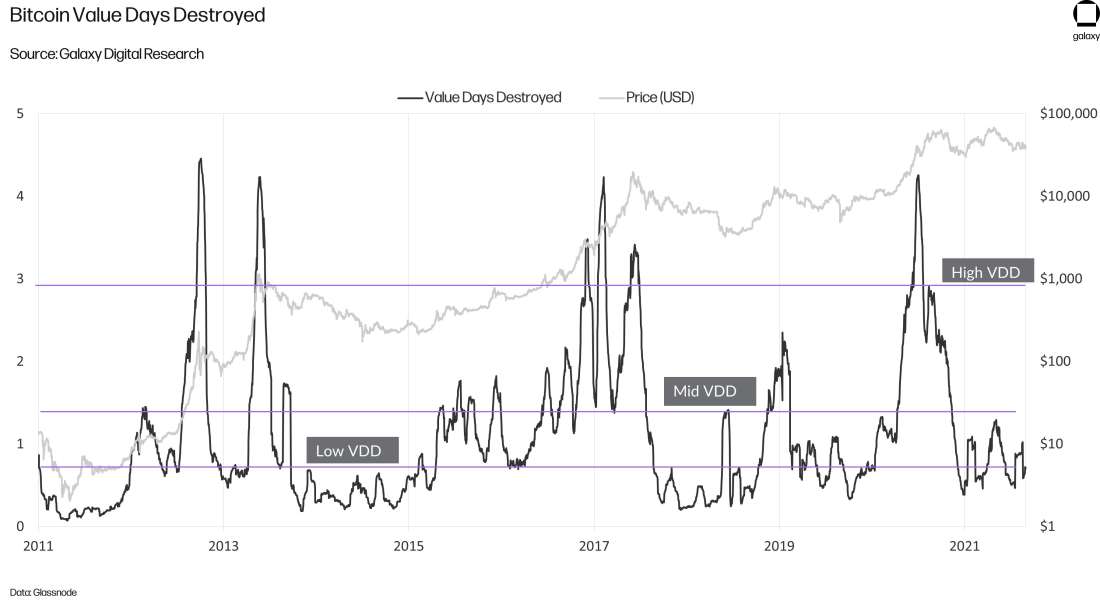
BDD and its related metrics are calculations inspired from the unique ways public blockchains offer researchers a window into saving and spending behavior. The next set of complex metrics that will be discussed are inspired from traditional metrics that are used for the fundamental analysis of public companies and equities.
Network Value to Transactions (NVT)
First introduced in 2017 by Bitcoin analyst Willy Woo, the Network to Value to Transaction (NVT) is modelled after the price-to-earnings (PE) ratio for valuing a traditional company.
The methodology for calculating NVT is: Market capitalization (USD) / Total transaction volume (USD).
Market capitalization is a metric that measures the outstanding value of a coin based on how many coins are in issuance (total supply) and the last known market price of a unit. There are separate ways to calculate market capitalization beyond the one shown below, which will be discussed in more detail later in this report.
The methodology for calculating market capitalization is: Price * Total supply.
NVT uses transaction volume as a proxy for measuring the utility users are deriving from the blockchain. NVT assumes that users are paying a non-negligible fee to send transactions on-chain, which, as discussed before, may not be true for certain general purpose blockchains such as Solana and Polygon. Normally, with a PE ratio, the denominator would measure the total earnings of a company but given that there are no earnings for a decentralized blockchain to report, transaction volume or in some cases, total fees, can be used instead.
The NVT ratio is often a lagging indicator of market bubbles, signaling periods when the market value of a coin is high relative to the coin’s underlying network utility. This can be caused if investors are valuing coins as a high return investment and especially when there exists a large degree of media hype or bullish market sentiment around a coin.
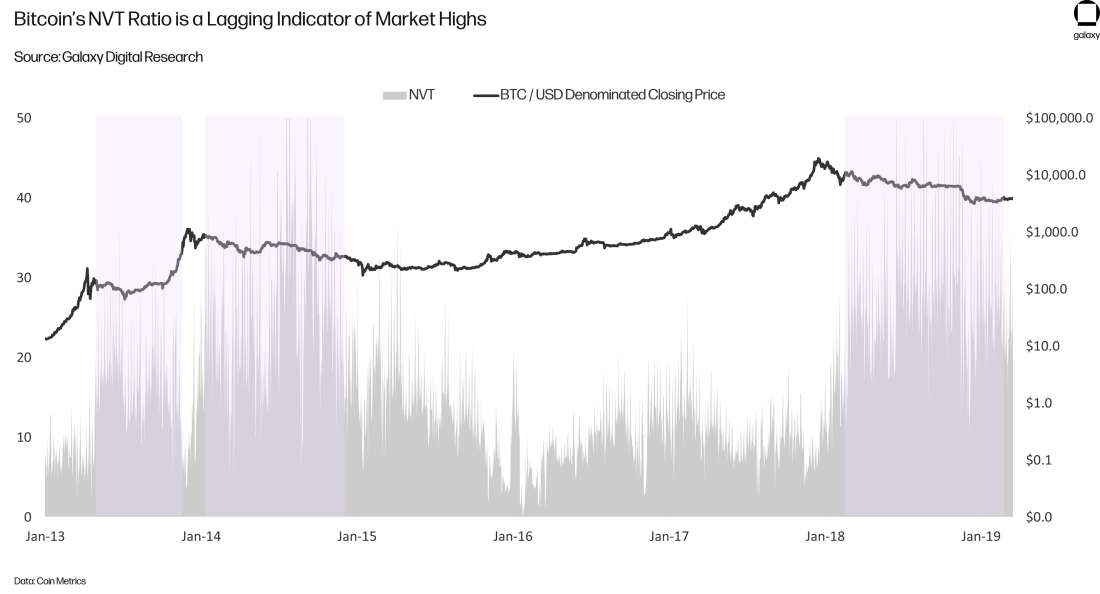
Given that peaks in NVT does not indicate or even coincide with market highs, alternative calculations for NVT try to better align movements in the metric with market cycles. The most used variation to NVT applies a moving average to the metric of transaction volume. A moving average helps smooth out irregularities in transaction volume and brings peaks of NVT closer in line with price action. The impacts of applying a longer moving average, such as a 90-day MA as opposed to 15 or 30-day, has been shown to be more effective in coinciding with price spikes.
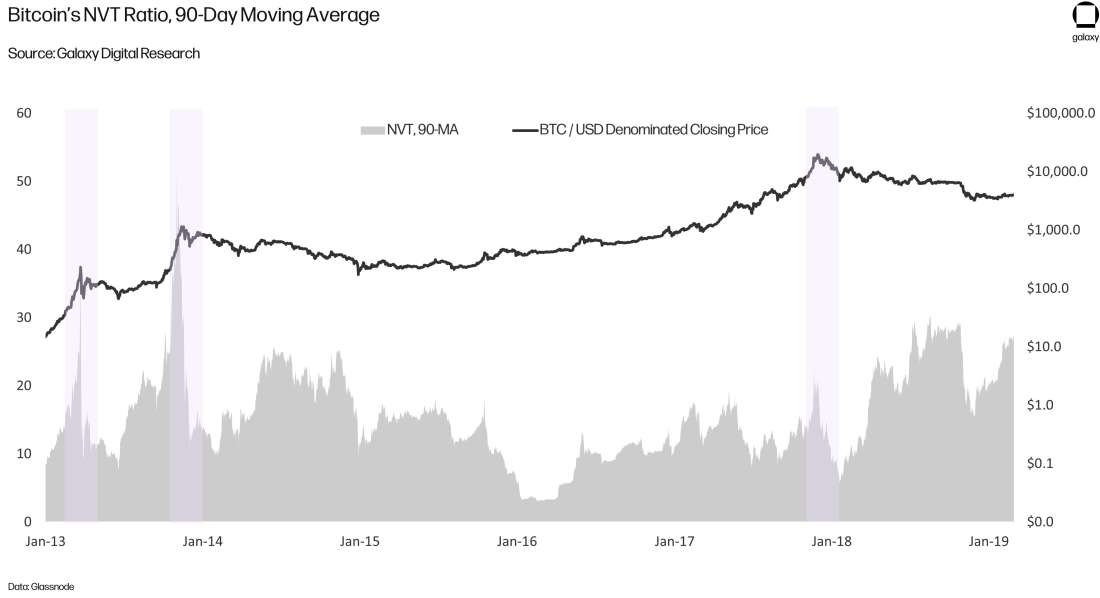
Modification #1: Changing the Building Blocks
In addition to applying moving averages, the basic metrics such as market capitalization and volume that make up NVT can be re-calculated to reflect on-chain activity more accurately. For example, adjusted transaction count can be used to calculate volume, which would help filter out some of the non-economic activity being transacted on-chain.
Similarly, NVT can also be re-calculated by adjusting the variable of market capitalization. Rather than using the traditional metric of total supply multiplied by coin price, market capitalization can be calculated by using a coin’s free float supply, which more accurately identifies supply that is available in the market to buy and sell.
The methodology for calculating free float market capitalization is: Price * Free float supply.
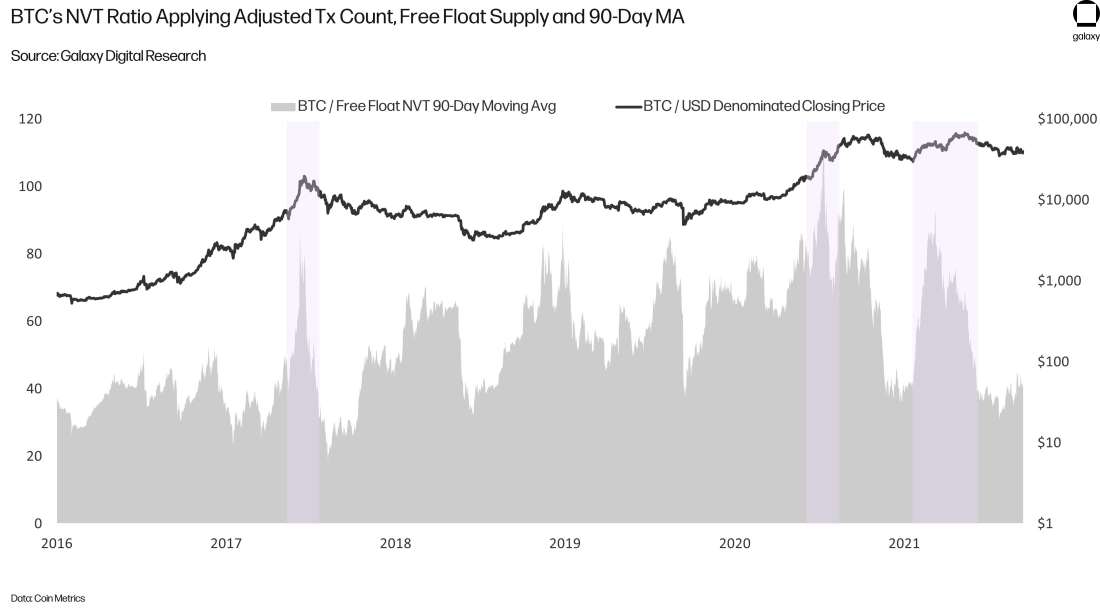
Modification #2: Alternative Complex Valuation
These adjustments as well as the basic concept for NVT have inspired a series of other complex metrics that focus on replicating traditional financial or economic metrics to cryptoassets. For example, a discounted cash flow (DCF) approach has been applied to cryptoassets like Bitcoin and Ethereum to determine a fair value for the asset in the hands of investors. DCF is traditionally calculated by projecting the year-end cash flows for an investment after relevant costs and expenditures are subtracted from revenue.
When applying even the most simplified and standard DCF calculation to Bitcoin, several variables do not transfer over such as taxes, depreciation, amortization, and other non-cash adjustments. When it comes to determining the operating expenses and capital expenditures of a cryptoasset like a traditional investment, the analysis becomes murky because of the varied ways in which various network stakeholders may contribute to the security and operations of a blockchain. However, some analysts have used DCF to argue the undervaluation of crypto assets such as ether. Using fees as the basis for calculating the revenue of Ethereum, and staking rewards, as well as burnt fees, as additional future cash flows, Ryan Allis of HeartRithm predicted in February 2022 a fair valuation of ETH according to a DCF model is $10,000 at a market capitalization of $4 tn.
Assuming Ethereum revenues grow 50 percent over the upcoming year and thereafter, at a decreasing rate down by 15 percent until 2035, Allis’ DCF model forecasts ETH price at $150,000 by the end of 2029. Now, there are several factors that may change this interpretation, including the rise of layer-two technology and sharding which is expected to significantly reduce the fees, and therefore the revenue, of Ethereum over the long-term.
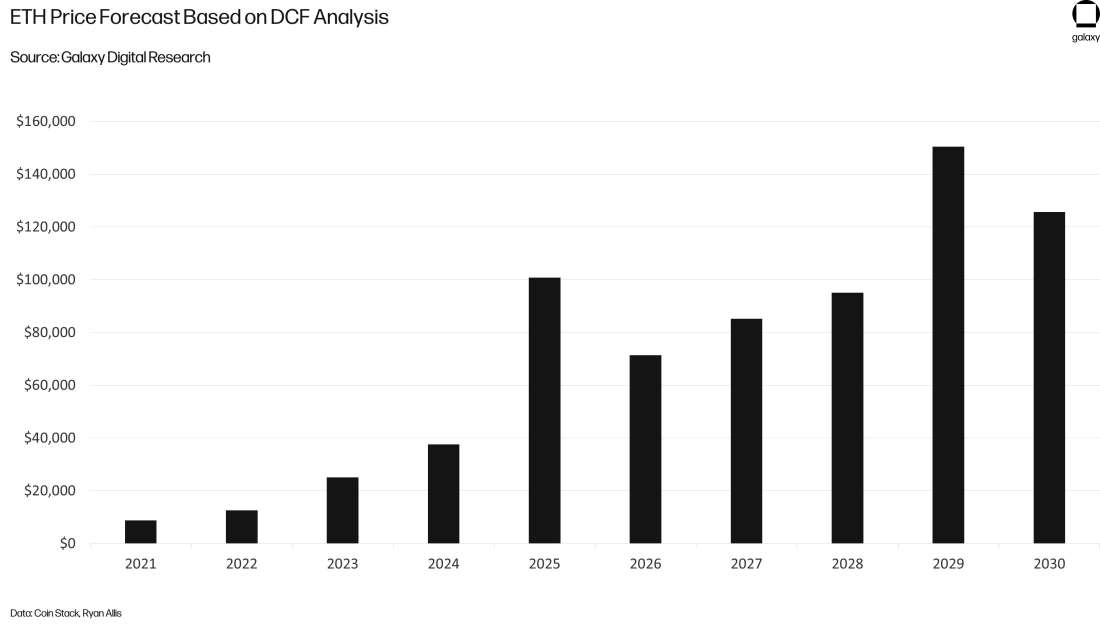
While DCF compares blockchains and their native crypto assets to traditional investments, other metrics such as coin velocity compares these networks to economies. Coin velocity looks at how quickly coins are exchanging hands between users. It is inspired by calculations for money velocity, which is used to identify expanding and contracting state economies.
The basic methodology for coin velocity is: Total value transferred / Current supply of coins.
There are limits to using transaction activity as a proxy for network utility depending on the use case of a cryptoasset. For proof-of-stake blockchains, most tokens are locked to provide security for the network and the lack of movement does not necessarily indicate a lack of utility for the token but rather could suggest greater confidence in the network as a security layer. In addition, bitcoin’s use as digital gold (i.e., a long-term store of value) has grown significantly over the past few years and is another example where coin velocity declined significantly but the value has not.
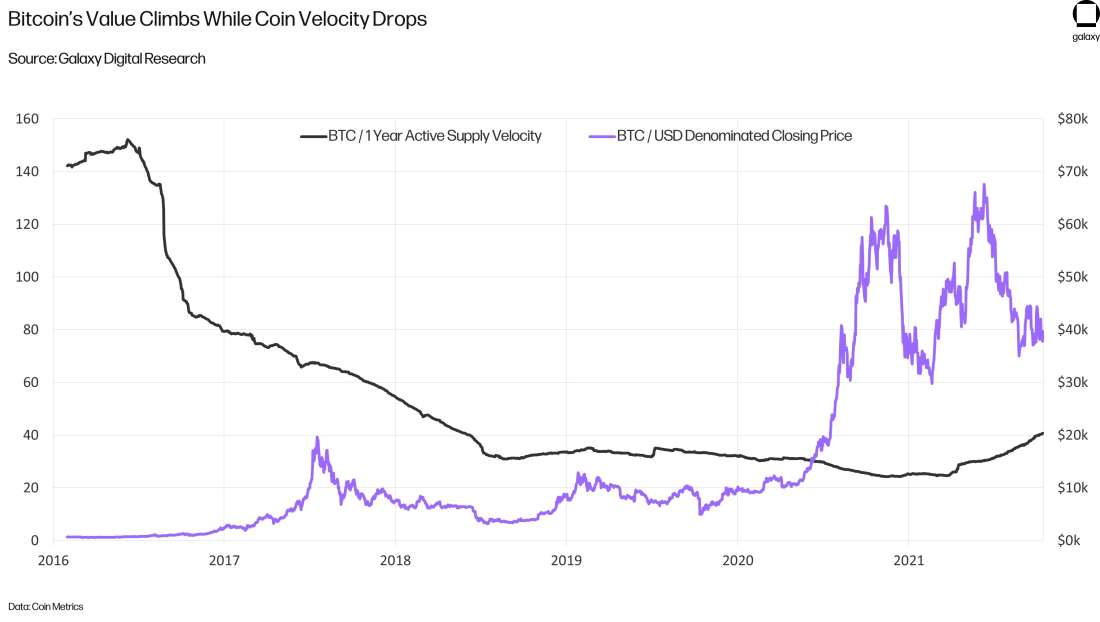
The comparisons between traditional company valuations and crypto, as well as national economies and crypto, are not one to one due to the plethora of reasons for why users are investing in these coins, which even for a single asset like Bitcoin, differs from holder to holder. However, from the viewpoint of a traditional investor, these types of analyses that seek to draw comparisons between cryptoassets and their underlying protocols as like that of a national economy or public company can act as a useful starting point for understanding the value cryptoassets.
Market Value to Realized Value (MVRV)
In October 2018, Bitcoin analysts Murad Mahmudov David Puell developed MVRV, which measures the amount of a coin’s supply that is held in profit versus held at a loss, on average.
The methodology for MVRV is: Market capitalization / Realized value.
Realized value is a metric developed by founder of blockchain data company Coin Metrics Nic Carter just before MVRV in September 2018 that builds upon BDD by extending the same logic to valuing the price of individual units of bitcoin. Instead of value all bitcoins in existence at the current market price, realized value evaluates and values each coin unit at the time it was last moved on-chain.
The methodology for calculating the network’s realized value is: SUM (Coin * Price at time last moved).
By taking the ratio of market capitalization to realized value, a researcher can get an idea of the average profitability of coin investors. If MV is higher than RV, this indicates on average investors are holding the coin at a profit. On the other hand, if MV is significantly lower, this indicates the average purchase price for all coins in existence is above what the market current values those coins at. Like many on-chain metrics based on coin movement, MVRV requires the researcher to assume that on-chain movement is indicative of coins changing owners.
Historically, for bitcoin, MVRV has usually trended above 1 suggesting Bitcoin has generally been a good investment.
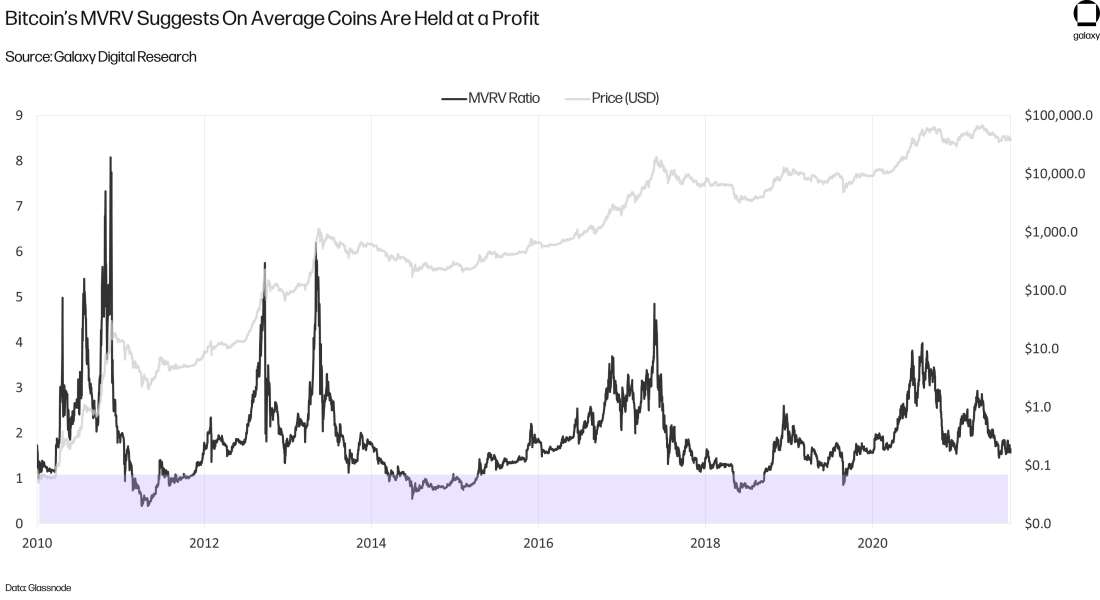
Modification 1: Changing the Building Blocks
A common variation of MVRV used to identify market peaks is the MVRV-Z score. Using the same variables, the MVRV-Z score indicates how far off the market value of an asset is from its realized value, relative to past movements in market value. In other words, this puts the difference in market value to realized value in context of historical swings in price.
The calculation for the MVRV-Z score is: (Market cap – Realized cap) / Standard deviation (Market cap).
When the MVRV-Z score is high, this indicates an unusually large gap between market cap and realized cap, which may tip off investors that a market correction is fast approaching.
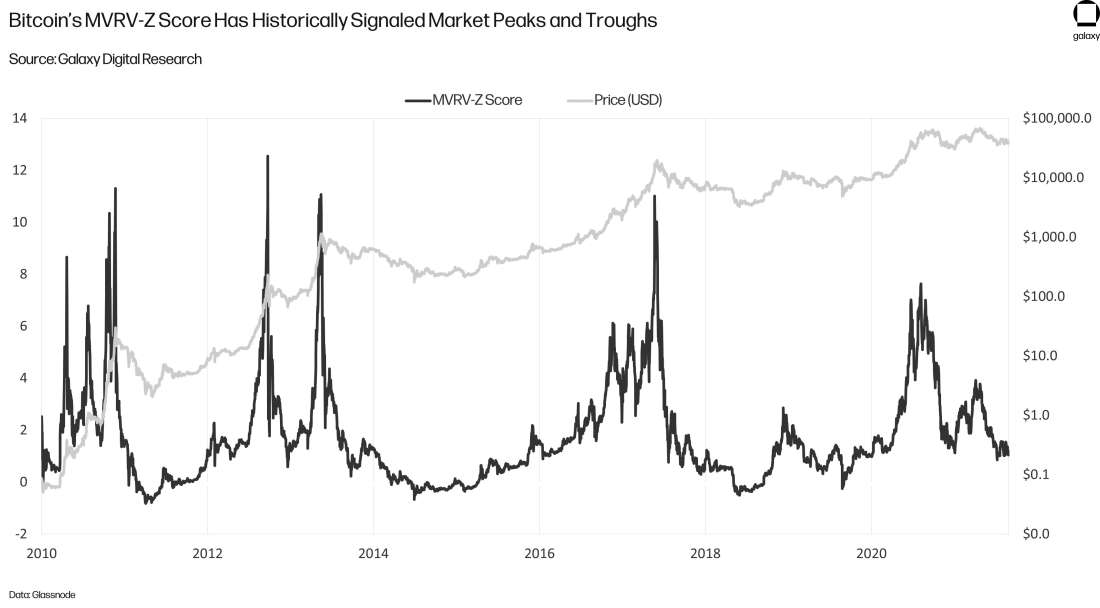
Another common Bitcoin-specific variation of MVRV is to calculate market capitalization and realized capitalization of only the coins that have not moved in more than some number of months (such as 5 months). This metric filters out younger coins and focuses on the value held by longer-term investors. Called the long-term holder MVRV (LTH-MVRV), this metric assesses the behavior of long-term investors and indicates when this group of investors are holding on average at a loss or a profit.
The methodology for calculating LTH-MVRV is: Market cap (of all coins that have not moved in more than 155 days) / Realized cap (of all coin that have not moved in more than 155 days).*
*While researchers can choose a different interval with which to filter MVRV, some data providers (like Glassnode) rely on 155 days as an important threshold that differentiates short-term and long-term coin holders.
Lastly, like BDD and NVT, MVRV can be adjusted through using alternative calculations for basic metrics such as market capitalization. Instead of using total coin supply, which as discussed may contain several coins that can no longer be bought or sold, free float supply is also frequently used to re-calculate market capitalization and adjust MVRV to better reflect activity in the available supply of a cryptoasset.
Modification 2: Alternative Complex Valuation Metrics
Building upon the ideas presented by MVRV, the Spent Output Profit Ratio (SOPR) captures the aggregate profit and loss of investors over the course of a day.
SOPR is calculated by: Realized cap (coins that have moved over the course of the day) / Market cap (coins that moved over the course of the day).
The goal of SOPR is to illustrate whether investors are selling off their coins at a loss or profit. As stated above, cryptoasset analysts will often use coin movement as a proxy for transfer of coin ownership, particularly on Bitcoin due to its lack of other, non-transfer-related on-chain uses for making capital productive, like DeFi. In bull markets, a larger portion of coins moving will be in profit. However, the SOPR will gradually decline as the bull market winds down and as the movement of coins at a loss continues to grow, the incentives for investors to hold their coins rather than sell also grows. This sequence of events can be identified in two stages:
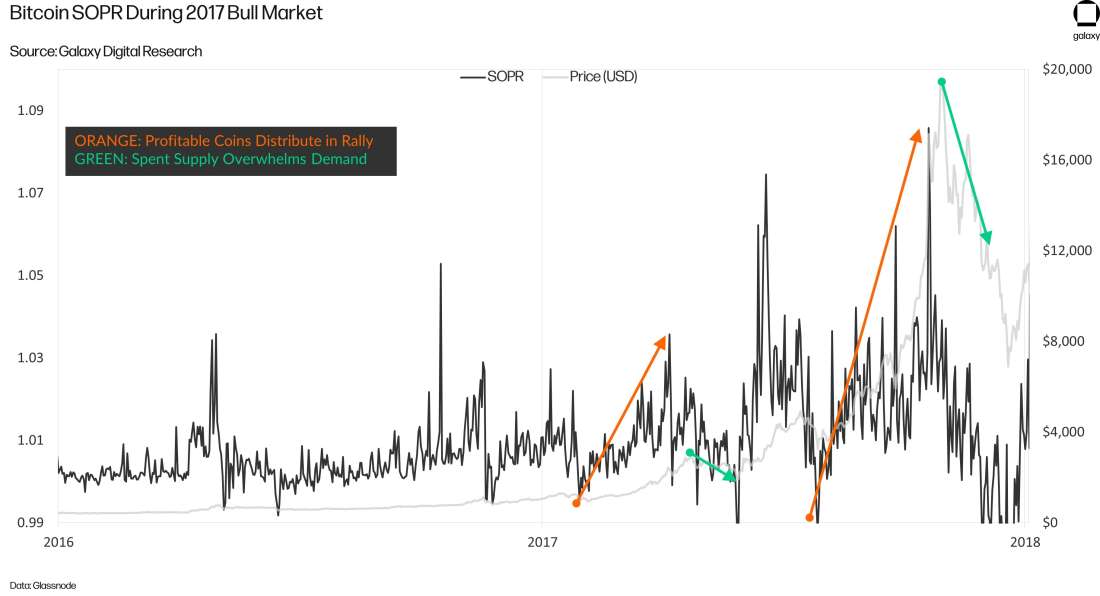
As indicated in the above chart, SOPR for Bitcoin has increased over time suggesting that coins have typically moved from short-term holders to those with longer time preferences over the course of multiple market cycles. Like MVRV, the methodology for SOPR can be adjusted to focus only on the activities of long-term and short-term coin holders. In addition, coins that move in rapid succession or have behavior that has a high likelihood of not being real economic activity can be filtered out. The adjusted SOPR methodology builds upon the adjusted transaction volume of coins mentioned earlier.
At this point, the variations of complex fundamental metrics should start to be repetitive and intuitive. Tools like free float supply and adjusted transaction volume are commonly used in on-chain analysis to filter out noise and tune into the real economic transaction activity of long-term and short-term holders of a coin. Before closing the discussion on SOPR, it’s also worth noting the other lenses through which profit and loss for a coin can be viewed.
Unrealized profit/loss is another related metric to MVRV and SOPR that looks at the delta between the price of a coin when it was created versus the current price of an asset. This may sound identical to MVRV but, in this case, the metric illustrates the outstanding profit to be made from all coins at any given time in the market.
It is calculated by: (Market cap – Realized cap) / Market cap.
This can be further adjusted by focusing on the unrealized profit or loss of long-term holders or short-term holders. It can also be re-calculated with alternative metrics such as free float supply as opposed to total supply. The metric has been used to evaluate market sentiment around a coin and has historically coincided with market peaks and troughs.
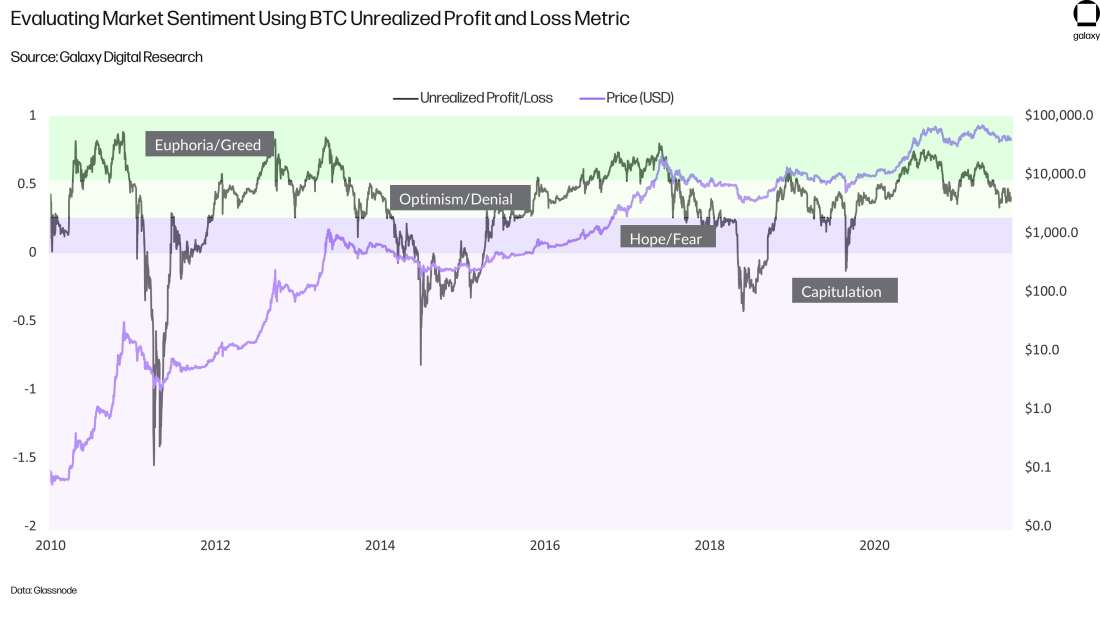
Relatedly, the entire supply of a cryptoasset can be recorded by the price at which each coin last moved to illustrate price band of buying and selling support. This is essentially realized capitalization but instead of shown in aggregate, it’s shown by price at time of last movement.
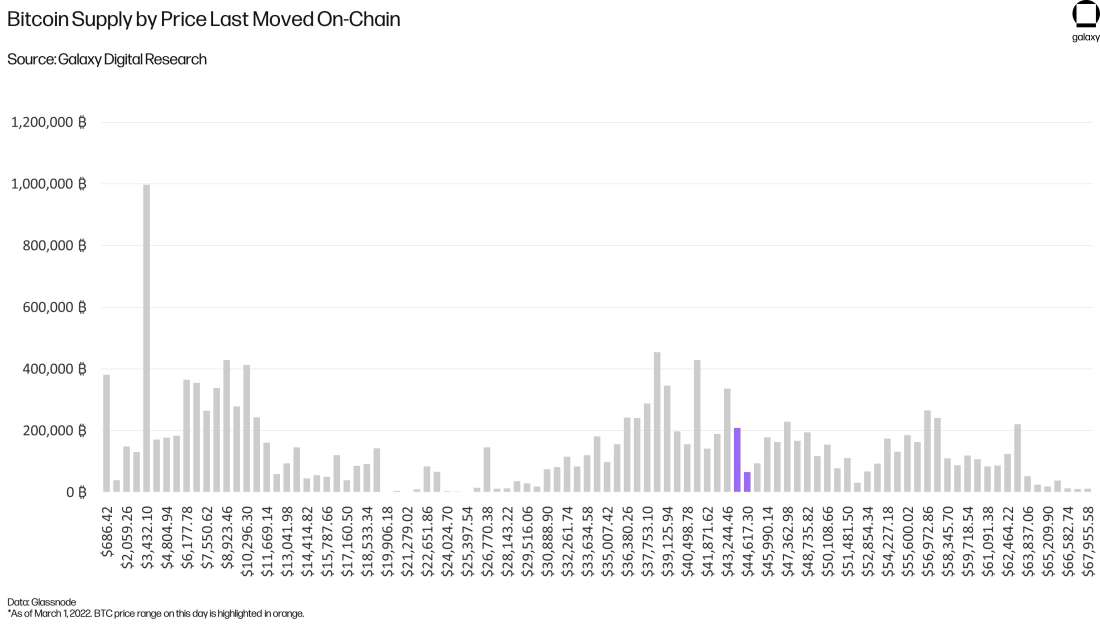
These kinds of analyses for evaluating the cost basis of investors have a narrow applicability when it comes to cryptoassets other than Bitcoin. Unrealized profit, SOPR, and MVRV are metrics that assume transaction activity is a proxy for investor trading behavior. While this may largely be true for cryptoassets like Bitcoin whose primary use case is that of store of value, this logic breaks down for other cryptoassets where on-chain activity is less likely to indicate a change of coin ownership.
On Ethereum, transactions are used to interact with decentralized applications (dapps) and transfers of value can be representative of smart contract activity, as opposed to trading activity. For example, a user may send funds to an automated market maker to earn fees from providing liquidity. In that instance, although the coins have transferred on-chain, their ownership has not. For newer blockchains such as Solana, Polkadot, and Avalanche, the transaction histories of these cryptoassets are limited and the assets have gone through fewer market cycles. A shorter data set for comparing on-chain data with market data is another factor making fundamental analysis harder on alternative cryptoassets to bitcoin. However, for each new blockchain, there are a new set of valuation metrics that are theorized to be potentially indicative of value.
Network-Specific and Application-Specific Metrics
Apart from basic on-chain metrics and complex metrics that can be modified to apply broadly to all cryptoassets, there are a slew of metrics inspired from network-specific activities and applications that can only be applied to a specific subset of cryptoassets.
Difficulty Ribbons
In 2019, bitcoin analyst Willy Woo (who also innovated the NVT metric) came up with a valuation model for Bitcoin based on miner activity. Difficulty ribbons are calculated by evaluating the moving averages of Bitcoin’s mining difficulty. Difficulty is a target set by the Bitcoin protocol that automatically adjusts every 2016 blocks, or roughly 2 weeks, to regulate the amount of computation needed to successfully mine a new block. Each calculation takes computational energy so the higher the number of hashes, the more computational energy required and the more difficult it is considered for miners to earn rewards.
In Woo’s incarnation of difficulty ribbons, he uses the 14-day, 25-day, 40-day, 60-day, 90-day, 128-day, and 200-day averages of mining difficulty to create a ribbon. Compressions of these averages, that is when the values are similar, suggests that competition between miners is decreasing. This is because a low variability across the moving averages indicates the mining difficulty is stabilizing as less efficient miners’ power down their machines.
Due to the energy intensive nature of proof-of-work mining, miners generally need to sell some amount of the bitcoin they earn to pay the recurring costs of their operations. (For more information about the operational costs of bitcoin miners, read this Galaxy Digital report on the cost of bitcoin mining.) As such, when the difficulty ribbon compresses and indicates miner capitulation, it also suggests a low sell pressure in the market and room for bullish price action.
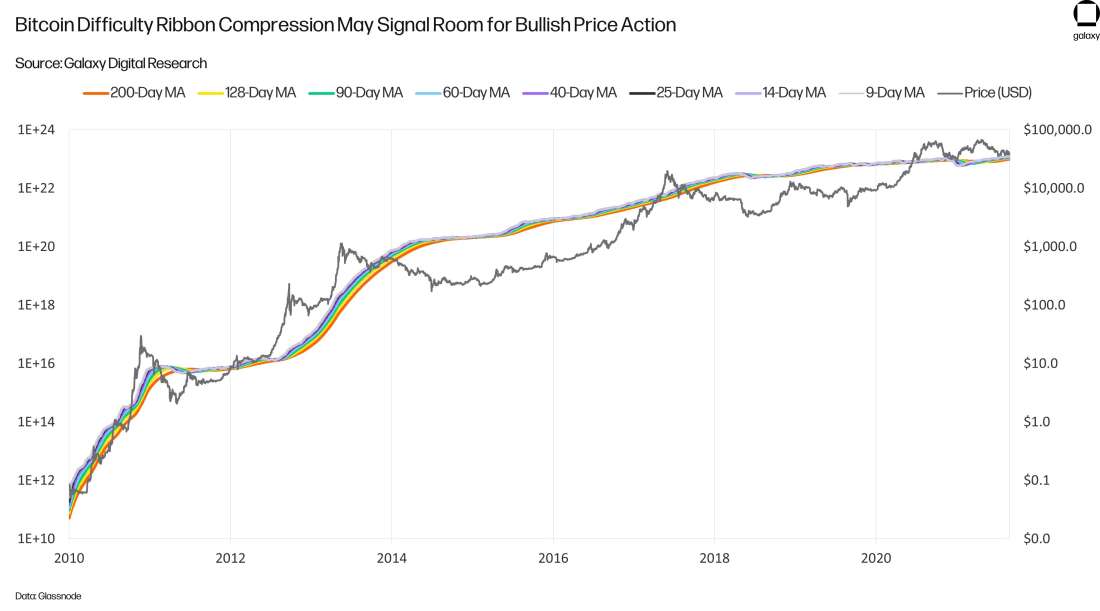
The difficulty ribbons are a valuation model for Bitcoin that only works because Bitcoin is based on a proof-of-work sybil resistance model. For proof-of-stake blockchains such as Polkadot and Avalanche, there are alternative metrics such as number of active validators and percentage of supply staked that can be used, though these are comparatively simpler and more straightforward.
Percentage of Total Supply Staked
One of the most common metrics used to evaluate the intrinsic value of cryptoassets that use proof-of-stake is the number of active validator entities. As opposed to miners, validators on PoS blockchain are responsible for validating transactions and creating new blocks. To be a validator, PoS blockchains require that users put up collateral also called stake in the form of native network tokens. For most PoS networks, the higher the number of validators, the higher the level of security for the network. However, there are blockchains such as EOS that intentionally cap the maximum number of validators that can be online at a given time.
Diving further into fundamental analysis for staking networks, the staked percentage of a coin’s total supply is another metric often used to evaluate PoS blockchains. A high percentage of staked coins is indicative of a large supply of illiquid coins locked by users to generate yield. Instead of block rewards, PoS networks reward validators for their stake through accrued interest on their locked collateral. There may be a predetermined vesting period for users to stake and un-stake their coins depending on the blockchain and the stage of the blockchain’s development.
The larger the number of coins that are staked and the longer the minimum duration that is required from validators, the smaller the supply of liquid coins becomes. If most coin holders are staking as opposed to interacting with dapps or transacting value peer-to-peer, this is suggestive that the PoS network’s primary use case is that of generating yield for validators.
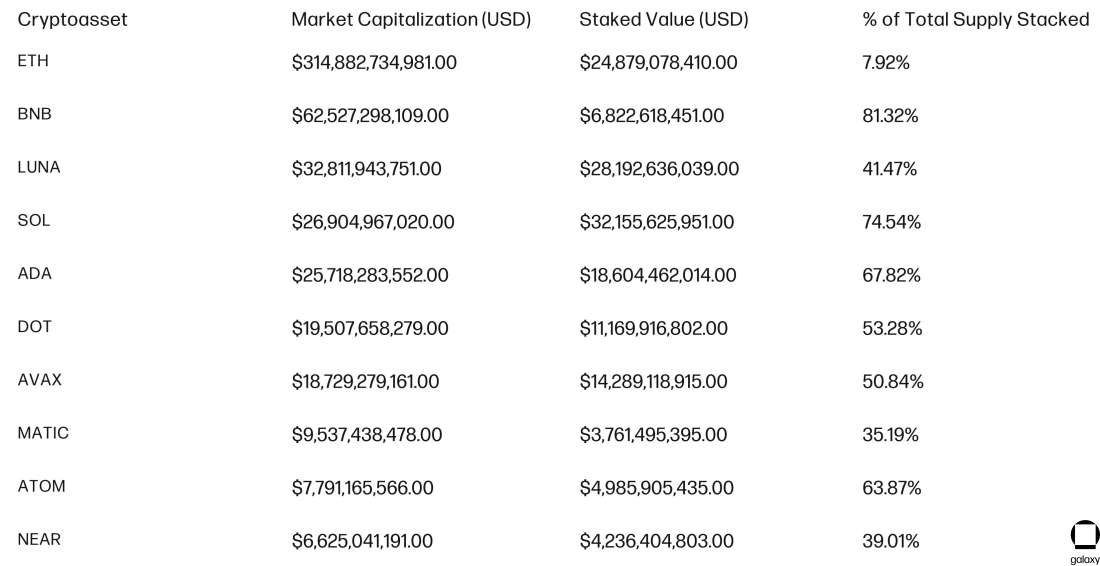
These metrics are not prescriptive or useful for understanding market movements like the other complex metrics discussed in this report. However, they do highlight important considerations about the intrinsic and long-term value of a coin’s fundamentals based on on-chain behavior. In conjunction with other on-chain metrics, fundamental analysis focusing on network-specific metrics are useful for understanding the behavior of a coin’s stakeholders beyond just investors or general users.
Total Value Locked
Decentralized finance (DeFi) and non-fungible tokens (NFTs) are two areas of innovation that has attracted investment and increased popularity since 2020. This has created a new class of fundamental analysis around these types of assets. A popular metric discussed for the fundamental analysis of DeFi protocol is total value locked (TVL). TVL, like total value staked, shows the amount of value managed by a smart contract protocol. TVL is typically used to describe the aggregate value locked in DeFi applications (in dollar terms) for the purposes of providing liquidity (in AMMs), earning yield (through yield farms or yield-farming aggregators), or lending pools. One benefit of TVL is that, because it's typically denominated in dollars rather than native units, is that it can be used to effectively compare DeFi activity across chains.
Innovations advancing fundamental analysis of DeFi protocols has led to the creation of metrics like the adjusted TVL metric. Due to price fluctuations that inflate or deflate the TVL of a DeFi protocol whenever the market moves as opposed to when users are newly adding or taking away collateral, adjusted TVL applies a simple moving average to asset prices so that TVL more accurately reflects real user activity.
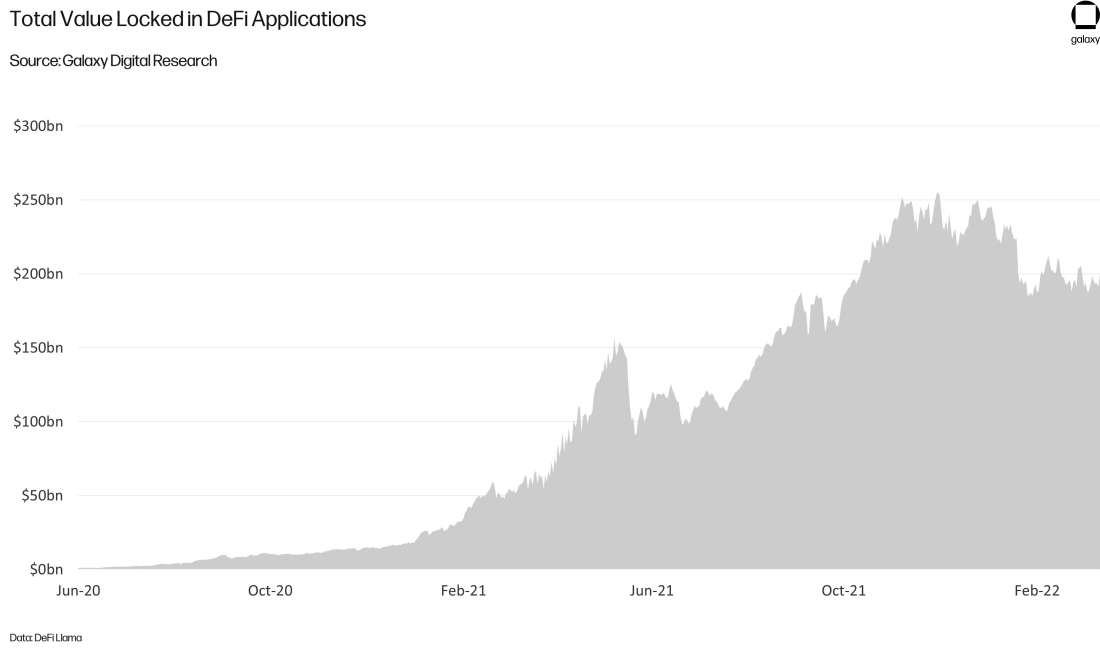
NFT Floor Prices
As the value behind NFTs grows, investors are also becoming increasingly attentive to on-chain metrics focused on crypto collectibles such the transaction count of ERC-721 and ERC-1155 tokens on Ethereum. Complex valuation metrics such as realized capitalization have also been modified for application to NFTs. Key to understanding how realized cap has been applied to crypto collectibles is the concept of an NFT floor price.
The floor price refers to the lowest sale price of an NFT within a specific collection. Though not all NFTs are part of a larger series, the ones that are such as the Bored Apes Yacht Club, Crypto Punks, Pudgy Penguins, Loot and more, are closely tied in value to the other NFTs issued within the same series. Within a collection, there may be rarer and therefore more valuable NFTs than others. However, overall, what impacts the value of a single Crypto Punk for example is likely to impact the value of other Crypto Punks in the same series.
Therefore, the floor price for an NFT collection is a useful indicator for generalizing the market performance of multiple crypto collectibles issued under the same project. In addition, NFT value may also be tracked using the metric of market capitalization using this idea of floor price. By multiplying the floor price with the number of issued NFTs in a collection, the lower bound for the value of the entire series can be calculated.
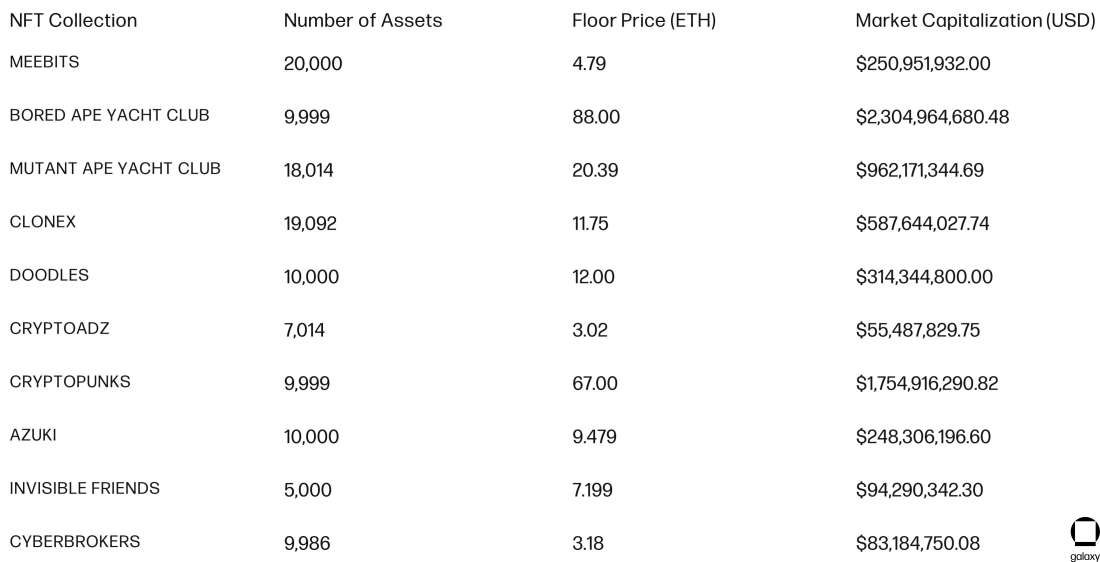
However, perhaps a more useful measure would be to price each NFT within a collection at the most recent price it was sold. This is like how fungible coins can be priced at the time they were last moved to measure the value of acquisition for these coins more accurately—essentially a measure of the aggregate cost basis of the collection. Applying this methodology to the Bored Ape Yacht Club collection, realized capitalization as of March 15, 2022, comes out to about $696mn, which is 70% lower than the quoted market capitalization of the collection. The realized capitalization of NFTs is often lower than the quoted market capitalization given that not all assets within a collection are actively traded or even yet minted.
As the adoption and value flowing through NFTs increase, fundamental analysis of these assets is also likely to mature. In this way, fundamental analysis of cryptoassets is an ongoing science and area of study that continues to evolve as new technologies and trends take hold within the crypto industry.
Conclusion
Since the advent of Bitcoin in 2009, tens of thousands of cryptoassets have been issued through myriad new blockchain protocols. Most fundamental analysis continues to center around Bitcoin, as it remains the world’s most valuable cryptoasset, but there are modifications for on-chain metrics like BDD and NVT that make them more applicable to other cryptoassets. With the intricacies and caveats discussed in this report in mind, investors can go about modifying and combining metrics to better understand the value of a cryptoasset. The next reports within Galaxy Digital’s Do Your Own Research series will build upon the framework discussed in this report to evaluate other sectors of the crypto industry.
Due to the diverse ways data providers can interpret blockchain data, it is important for investors to start learning fundamental analysis by first understanding the popular methodologies for calculating on-chain metrics. Even for a basic on-chain metric such as coin supply, the methodology used can drastically change the resulting values. In addition, for more complex metrics such as difficulty ribbons, the methodology may only be applicable to a specific subset of cryptoassets. Most complex valuation models are extremely limited when it comes to their universality and applicability between different cryptoassets.
In addition, on-chain metrics in general do not capture the investment activity of network stakeholders occurring off-chain, such as on cryptocurrency exchanges, custodial payments applications, and even layer-two networks such as the Lightning Network. Activity occurring off-chain is outside of the scope of on-chain fundamental analysis, which is why supporting on-chain data with data from other sources is also an important consideration for drawing nuanced conclusions about cryptoasset valuation. Still, knowing these valuation models helps create a framework for understanding how on-chain data can be used to uncover important trends about a cryptoasset’s value.
Public blockchain data is a powerful and unprecedented tool when it comes to the field of fundamental analysis in finance more broadly that offers investors complete transparency into the use of a public blockchain network. Though metrics can be easily misinterpreted if applied without context, there are ways to contextualize on-chain data through applying filters, as well as alternative methodologies, for investors to begin valuing cryptoassets more accurately. On-chain data is essential for tracking the usage of public blockchains and, moving forward, fundamental analysis based on on-chain data will become an increasingly popular and necessary tool for evaluating the value of cryptoassets and understanding their market movements alongside technical and social sentiment analyses.
Legal Disclosure:
This document, and the information contained herein, has been provided to you by Galaxy Digital Holdings LP and its affiliates (“Galaxy Digital”) solely for informational purposes. This document may not be reproduced or redistributed in whole or in part, in any format, without the express written approval of Galaxy Digital. Neither the information, nor any opinion contained in this document, constitutes an offer to buy or sell, or a solicitation of an offer to buy or sell, any advisory services, securities, futures, options or other financial instruments or to participate in any advisory services or trading strategy. Nothing contained in this document constitutes investment, legal or tax advice or is an endorsementof any of the digital assets or companies mentioned herein. You should make your own investigations and evaluations of the information herein. Any decisions based on information contained in this document are the sole responsibility of the reader. Certain statements in this document reflect Galaxy Digital’s views, estimates, opinions or predictions (which may be based on proprietary models and assumptions, including, in particular, Galaxy Digital’s views on the current and future market for certain digital assets), and there is no guarantee that these views, estimates, opinions or predictions are currently accurate or that they will be ultimately realized. To the extent these assumptions or models are not correct or circumstances change, the actual performance may vary substantially from, and be less than, the estimates included herein. None of Galaxy Digital nor any of its affiliates, shareholders, partners, members, directors, officers, management, employees or representatives makes any representation or warranty, express or implied, as to the accuracy or completeness of any of the information or any other information (whether communicated in written or oral form) transmitted or made available to you. Each of the aforementioned parties expressly disclaims any and all liability relating to or resulting from the use of this information. Certain information contained herein (including financial information) has been obtained from published and non-published sources. Such information has not been independently verified by Galaxy Digital and, Galaxy Digital, does not assume responsibility for the accuracy of such information. Affiliates of Galaxy Digital may have owned or may own investments in some of the digital assets and protocols discussed in this document. Except where otherwise indicated, the information in this document is based on matters as they exist as of the date of preparation and not as of any future date, and will not be updated or otherwise revised to reflect information that subsequently becomes available, or circumstances existing or changes occurring after the date hereof. This document provides links to other Websites that we think might be of interest to you. Please note that when you click on one of these links, you may be moving to a provider’s website that is not associated with Galaxy Digital. These linked sites and their providers are not controlled by us, and we are not responsible for the contents or the proper operation of any linked site. The inclusion of any link does not imply our endorsement or our adoption of the statements therein. We encourage you to read the terms of use and privacy statements of these linked sites as their policies may differ from ours. The foregoing does not constitute a “research report” as defined by FINRA Rule 2241 or a “debt research report” as defined by FINRA Rule 2242 and was not prepared by Galaxy Digital Partners LLC. For all inquiries, please email [email protected]. ©Copyright Galaxy Digital Holdings LP 2022. All rights reserved.